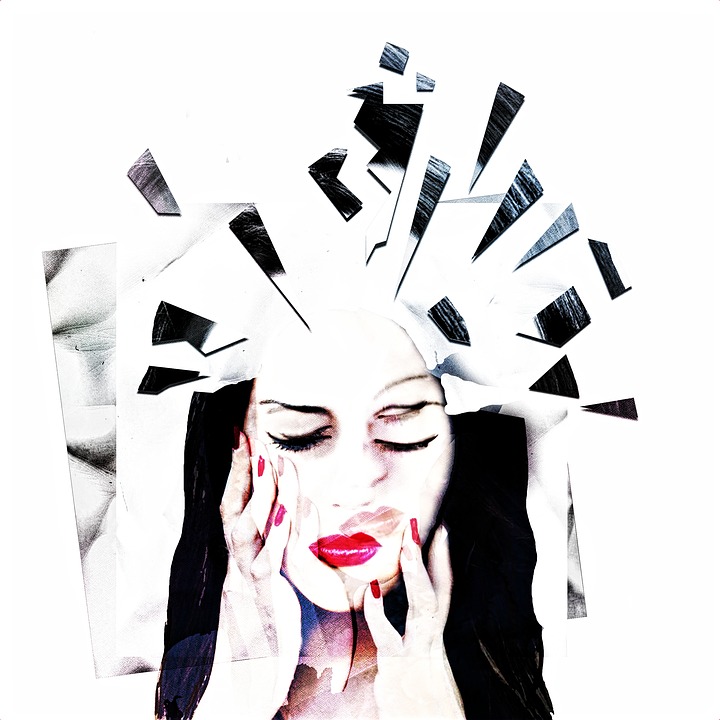
Machine learning techniques have been experimented with to assist the diagnosis of conditions like autism and epilepsy. Now, forms of psychosis-like schizophrenia can be added to that list. Recently, researchers from Harvard University and Emory University developed a machine learning-based method for predicting the probability of the emergence of psychosis in an individual’s future. The method analyzes the speech an individual uses and tracks how frequently words associated with sound are used. When combined with a method for quantifying an individual’s semantic richness in everyday language, the machine learning system can predict if an individual will later develop schizophrenia with approximately 93% accuracy.
The machine learning model relies on two different language-based variables or features, as EurekaAlert reported. The first feature is the frequency that words associated with sound manifest within a person’s language, and the second feature is the “semantic richness” of an individual’s speech, or how often they speak phrases with different semantic meaning. If an individual has a high usage of sound associated words and low semantic density (vagueness), the person in question will later manifest some type of psychosis.
Developing Models To Analyze Language
The research is surprising in part because although hearing abnormal sounds is a pre-clinical symptom of schizophrenia, many trained psychiatrists or clinicians had not picked up on the fact that people at risk for psychosis frequently used sound-related words, more so than the average person.
As reported by EurekaAlert, the paper’s first author, Neguine Rezaii, explains that the power of the machine learning-based method is that it can detect linguistic patterns, patterns which, despite their relevancy, go unnoticed by the vast majority of people. Rezaii says that trying to distinguish the subtleties of word usage by yourself is “like trying to see microscopic germs with your eyes”, but that the machine learning method is “like a microscope for warning signs of psychosis.”
Computers are capable of processing much more information than a human can, and machine learning systems excel at extracting patterns from large databases of occurrences. While the linguistic features that predicted psychosis were always present in people’s language, even trained clinicians were not able to make effective predictions about psychosis based off of these features, as these patterns were obscured in the massive amount of data (the words a person may use in conversation) they saw on a daily basis. The machine learning techniques that the authors used were capable of uncovering the hidden patterns within those features.
The research team utilized machine learning algorithms to create a baseline of conversational language they could compare abnormal linguistic patterns against. The data fed into the machine learning model was pulled from conversations from approximately 30,000 users of Reddit, the internet news board and social media forum. The researchers made use of a group of natural language processing models called Word2Vec, developed by a team of researchers from Google.
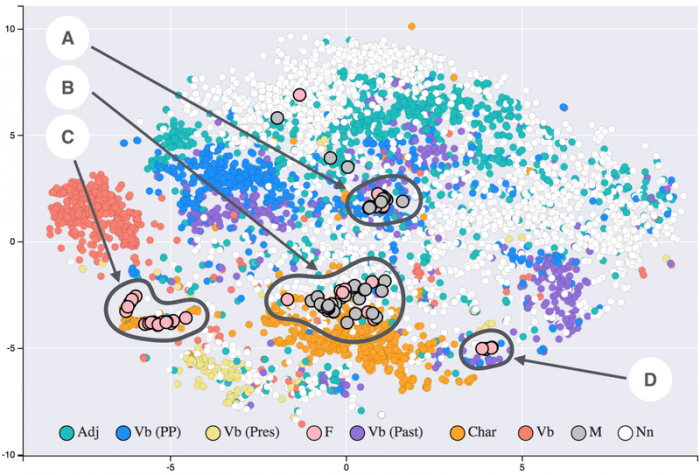
Photo: (https://commons.wikimedia.org/wiki/File:T-SNE_visualisation_of_word_embeddings_generated_using_19th_century_literature.png) by Siobhán Grayson via Wikimedia Commons, CC BY SA 4.0 (https://creativecommons.org/licenses/by-sa/4.0/deed.en)
The Word2Vec models produce word embeddings, which are mathematical vectors filled with real numbers. Machine learning models cannot work with natural human text, so the text must be converted to numbers in order for the algorithms to interpret them. Word embedding techniques like those used in Word2Vec not only create vectors of real numbers, they represent these vectors within a matrix. Similar words will have similar vectors within the matrix, with the distance between word vectors increasing as the words become less similar. Because words with different meanings will be position farther away while words with similar meanings are positioned closer together, it is possible to discern trends in an individual’s speech. For instance, words that relate to sounds should be closer to each other within the space of word vectors than non-sound related words.
The researchers also created a program that would analyze the semantic relationships between words used in sentences, quantifying the semantic density of words used in a process known as “vector unpacking”. Vector unpacking allows data analysts to quantify how much information is conveyed by a given a sentence, and when these vector unpacking methods are combined with Word2Vec models, “normal” semantic representations can be compared to “abnormal” semantic representations.
The researchers used the relevant data to create a series of normal word vectors to compare the speech of individuals with high risk of psychosis against. The researchers created word vectors generated from interviews of 40 different participants that had been conducted by mental health professionals. These interviews were funded by the National Institutes of Health and part of the North American Prodrome Longitudinal Study (NAPLS), which tracked adolescents with a high risk for developing psychosis.
The data generated from the NAPLS participants were compared to the normal baseline samples, using longitudinal data on whether or not the participants were eventually diagnosed with psychosis as context. When the speech patterns of these individuals were compared to the normal speech patterns, it was found that a higher than normal usage of sound related words, as well as a predisposition to use words with highly similar meanings, was highly suggestive of later manifestation of psychosis.
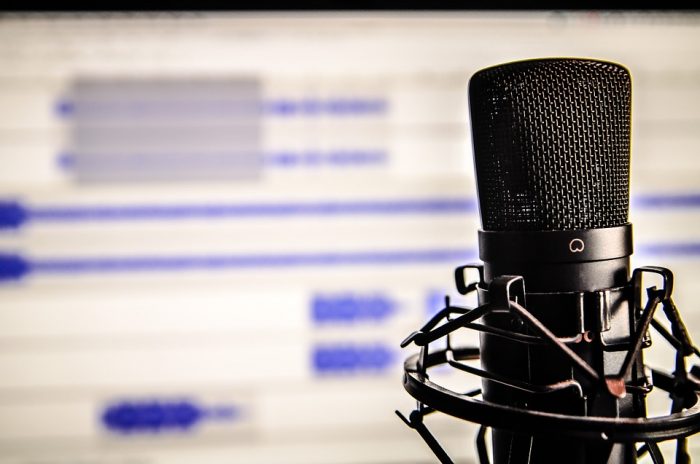
Photo: (https://pixabay.com/photos/microphone-audio-computer-338481/) via TheAngryTeddy (https://pixabay.com/service/license/)
The study utilized just two variables, sound-related words and semantic richness, and both of these variables are highly correlated with the development of psychosis, having a robust theoretical foundation. The model also replicated the results when tested on a hold out data set, and it achieved a high predictive accuracy, above 90% accuracy total.
Future Applications
Elaine Walker, Emory professor of neuroscience and psychology, and co-author on the paper explained to Emory University’s eScience Commons that their research demonstrates the potential for machine learning systems to “identify linguistic abnormalities associated with mental illness”.
According to mental health research, psychotic disorders like schizophrenia typically manifest themselves in a person’s early 20s, with indicators and warning signs developing around 16 or 17. These warning signs are known as prodromal syndrome. Somewhere between 25% to 30% of adolescents who have prodromal syndrome will later develop a psychotic disorder like schizophrenia.
Trained clinicians can correctly predict the development of psychosis around 80% of the time when the individual in question has prodromal syndrome, and they base their predictions on the individual’s performance at a series of cognitive tasks and structured interviews. Machine learning techniques can be used to create assistive tools that help clinicians diagnose patients with greater accuracy, extracting the relevant features and identifying variables that even trained professionals have difficulty identifying.
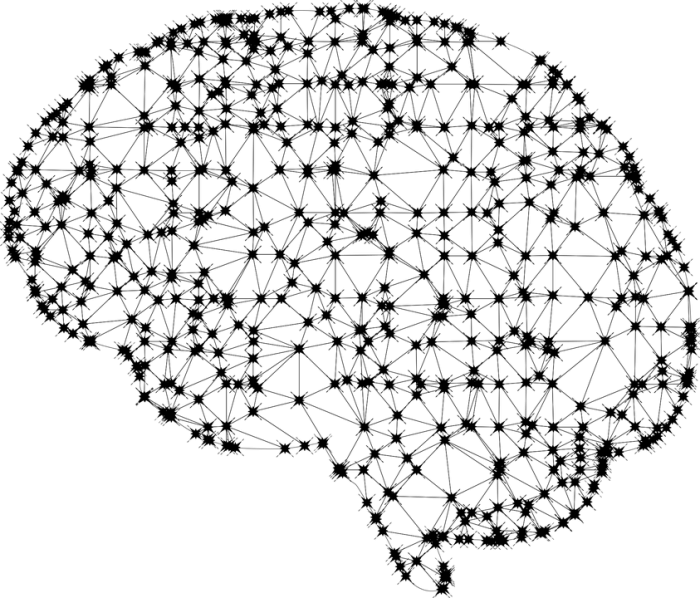
Photo: (https://pixabay.com/vectors/a-i-ai-anatomy-2729794/) by GDJ via Pixabay, Pixabay License (https://pixabay.com/service/license/)
There are many possible benefits to improving the accuracy of psychosis predictions. While there is no known cure for psychosis at the moment, psychosis can be treated. Walker explains that machine learning algorithms could potentially identify at risk individuals earlier in the timeline of the disease, and with these early warning signs comes the chance to use interventions that could reduce the severity of the disease or reverse deficits.
“There are good data showing that treatments like cognitive-behavioral therapy can delay onset, and perhaps even reduce the occurrence of psychosis, ” said Walker to eScience Commons.
The research team is now in the process of gathering more datasets and trying to expand the scope of their methods to other neuropsychiatric illnesses. They hope that the methods could have a similar predictive power for mental illnesses like dementia.
Philip Wolff, the senior author on the paper and professor of psychology at Emory University, explained to EurekaAlert that machine learning technology is creating new tools to data mine the human mind.
Other Machine Learning Applications For Mental Health
The research conducted at Emory University of Harvard University isn’t the only recent application of machine learning techniques in the field of mental health. Two mental health-based startups have invested in apps that would act as early warning systems for those who struggle with anxiety-related disorders and have frequent emotional crises. Machine learning techniques have also been tested for their ability to predict postpartum depression.
As Gizmodo reports, the state of California has been in talks with two different startups to create a system for smartphone users that will track a user’s behavior and alert them to a possible emotional crisis. The two startups are 7 cups, an online based therapy provider, and MindStrong which has developed an app that predicts one’s moods based off of their screen activity. MindStrong recently ran a trial where a custom keyboard was installed to the user’s phone, and this keyboard analyzed the screen activity of the user. If many instances of divergent behavior are detected by the keyboard app, the app will notify the user that they are potentially experiencing an emotional crisis. While there are privacy concerns associated with the MindStrong app, and similar apps, there is a potential for genuine benefit as well, in the creation of a system that can prompt vulnerable people to seek help in moments of distress.
Finally, according to PyschCongress, machine learning has demonstrated potential to predict the development of postpartum depression, by analyzing the common kinds of data collected by health officials during meetings with patients. The machine learning models are trained on features such as prenatal mental health, infectious disease diagnosis, third-trimester pelvic pain, third-trimester vitamin intake, abdominal pain, ethnicity, and age, all variables that have been associated with postpartum depression. The researchers hope they can improve the predictive power of their models by including information about drug exposure during prenatal care as well as disease history