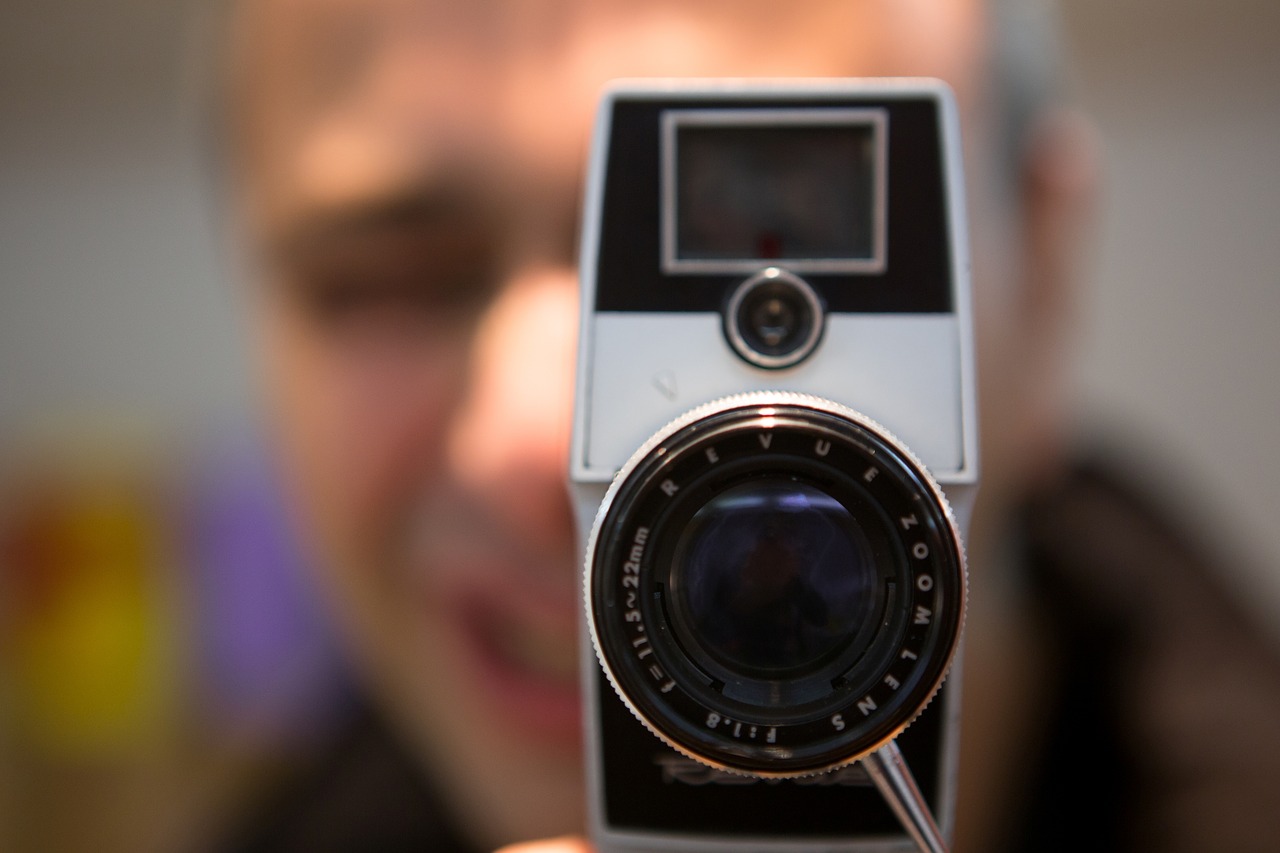
Autism Spectrum Disorder is one of the fastest growing developmental delays and has risen by 700% since 1996, now impacting 1 in 40 children in the United States. This causes a significant financial burden on families, with individual costs going up to $60,000 per year to support someone diagnosed with autism.
The current method of diagnosis for autism is based on observation and parental interview and involves several in-person appointments with trained clinicians who measure between 20-100 behaviors. These appointments can last several hours and therefore, with the growing demand for diagnostic resources for autism, have resulted in long waitlists. This causes a significant delay in diagnosis for several children, and, in some cases, the children are not diagnosed until after the age at which interventions can be most effective.
Through machine learning and large scale data mining, the Wall Lab, with Principal Investigator Dr. Dennis Wall, has built a mobile system that quantifies and tracks the severity of autism spectrum disorder. This can be a scalable, low-cost, and effective solution to break the diagnosis bottleneck and have a global impact across cultures and different development delays.
Standard diagnostic instruments for autism include the Autism Diagnostic Observation Schedule (ADOS) and the Autism Diagnostic Interview-Revised (ADI-R). In previous work, Wall’s team collected clinical scoresheets from ADOS and ADI-R and by performing feature reduction, determined the minimal number of features required in order to reach an accurate diagnosis. This work has resulted in 8 unique machine learning models, several of which have been independently validated by the Wall Team using external data as well as by other researchers using distinct cohorts of children with and without autism, showing balanced measures of accuracy consistently above 80% and often above 90%. In aggregate these models use 30 features that the Wall group hypothesized could be measured, or “tagged,” accurately by untrained individuals in a short home video under 3 minutes in length and often as short as 60 seconds, depicting the child at risk in his or her natural setting.
The video raters, chosen deliberately to be non-expert, namely without explicit training in the field and not certified for clinical practice in developmental delay detection, were given minimal video feature analysis training by the Wall Lab’s clinical managers and the task of accessing the videos through a secure portal and rating the 30 features on 162 videos of American children. The features tagged by these nonexpert video analysts included social interaction, interaction with objects, and speech-language traits. The videos were collected through online crowdsourcing or through public data sources such as YouTube. The independent tagging measurements generated by nonexpert raters on the 30 features were delivered as input to 8 validated classifiers in order to prospectively validate them on this new feature set from video data. The features being input to these classifiers were different from the features they were trained on for various reasons.
The Wall team tested and determined that three video raters, blind to the diagnosis and working independently on each video, would be sufficient to reach majority rules consensus on the diagnosis for the child in question. With this minimally viable “crowd” of nonexpert raters, the Wall team ran a prospective home video analysis on 116 children with autism ages 2-8 and 46 neurotypical children ages 2-6. The median time to complete the 30 feature tagging task was 4 minutes, resulting, in that short period of time, in 3 unique and independent feature vectors enriched for the child’s natural behavior for use in model computation.
Although several models performed well, a sparse 5-feature Logistic Regression classifier yielded the highest accuracy of 92% across all ages tested, importantly including children at and under 2 years of age. The Wall team then used a separate and independent prospective dataset of 66 videos (33 ASD and 33 non-ASD) with the same 30 features tagged on them by 3 new nonexpert raters’ measurements to validate the outcome, achieving slightly lower but still comparable accuracy at 89%.
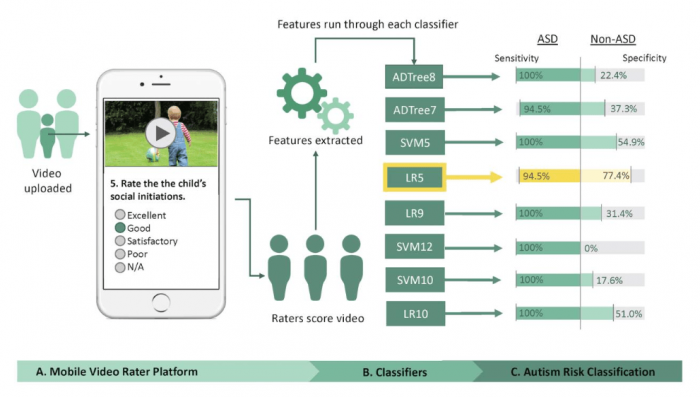
Image republished from PLOS Medicine from https://doi.org/10.1371/journal.pmed.1002705
This mobile machine learning process for diagnosis can accelerate the detection and enable triage to ameliorate the diagnostic bottleneck but can also instantiate an “action-to-data” feedback loop by enabling point-of-care diagnostics at scale while building feature labels on images that can be used later for deep learning model development. Such labeled image data could have even greater value for detection and tracking of autism over time. The work also suggests that non-experts working virtually — a crowd workforce — may enable rapid and economical feature analysis without compromising accuracy. Compliance, structure, and volume are essential for scaling video analysis of the kind described here to the global population in need of initial detection and in need of being quantitatively tracked during therapy. The Wall Lab project Guess What is a mobile video game that encourages and records social interactions between child and caregiver enriched for the features needed by our classifiers. As a record-while-you-play style of game for both Android and iOS, it has the potential to facilitate video collection at high volume with little to no technical barriers. For developing countries, this screening system may be particularly useful.
To this end, the Wall lab has recently completed the first phases of a follow-on project, recently published in JMIR, which shows the ability for the mobile video and machine learning processes to work on children at risk for a host of developmental delays, well under 4 years of age and native to Bangladesh, a developing country with few pediatric health resources. This expansion and adaptation of the prior work show that such an approach is culturally scalable, can help with the diagnosis of other developmental delays, and provide a vehicle for mapping the prevalence of developmental disorders across the country.
These findings are described in the article entitled Mobile detection of autism through machine learning on home video: A development and prospective validation study, recently published in the journal PLOS Medicine.