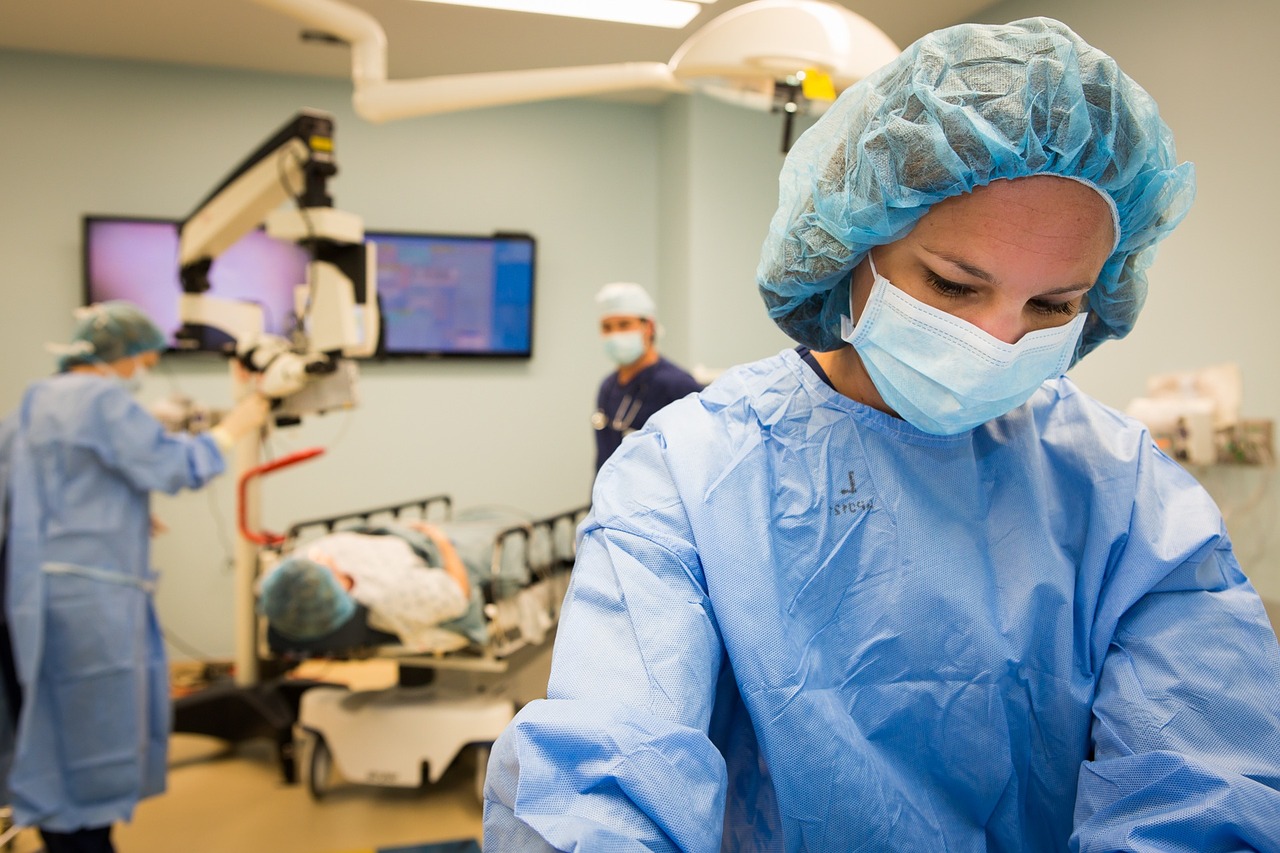
In today’s world, advanced technology has been embedded in almost every aspect of life. Machine learning, a type of statistical artificial intelligence (AI), is no exception. From Andrej Karparthy’s Tesla AI system to Stitchfix’s algorithms team, successful companies have incorporated machine learning and data science into business plans for automation purposes. However, this trend has been slow to reach the health sector. Due to difficulties in access to healthcare data, complex workflows, stringent regulatory oversight, and the measured adoption of innovation, cutting edge technology can have a challenging time getting its foot in the door.
Seeing machine learning’s potential through its gains in other industries, the academic healthcare community is slowly accepting data science and machine learning as a new way to improve care. At the Duke Institute for Health Innovation, our team works to apply machine learning to the healthcare space in a way that can help support patient-physician decision making. Last year we developed 14 postoperative complication risk prediction models1 in order to better inform patients and physicians before surgery about potential health risks that might occur after surgery such as death, infections, bleeds, and other serious complications. These models were trained on electronic health record data from a cohort of 90,145 patients that had undergone 145,604 invasive surgeries. The models use 194 patient data features, representing a patient’s past and current health, to make predictions about how a patient is likely to successfully recover from an operation.
We built these models using three different types of machine learning methods: LASSO, random forest, and extreme gradient boosted decision trees. These three methods were utilized in order to compare model performance and determine the optimal statistical method (Figure 1).
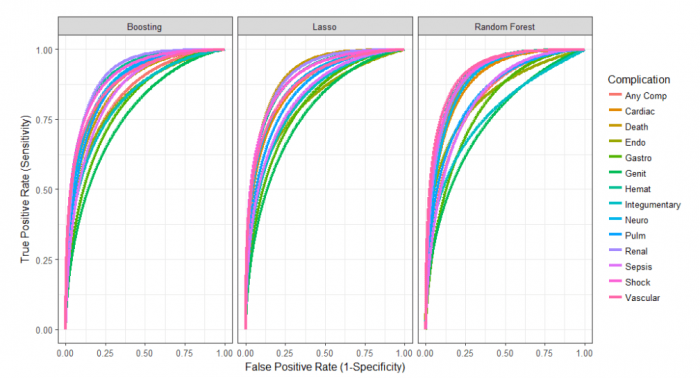
Image republished from PLOS Medicine from https://journals.plos.org/plosmedicine/article?id=10.1371/journal.pmed.1002701, licensed under CC-BY 4.0
Due to LASSO’s superior performance and clinical interpretability, we designed a simple calculator around the LASSO models for easy usability in preoperative clinics so that physicians can input patient information and receive additional data concerning whether patients’ are at high risk of postoperative complications (Figure 2).
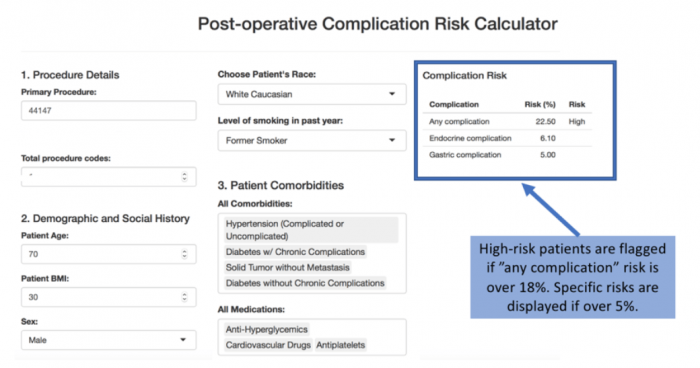
Image republished from PLOS Medicine from https://journals.plos.org/plosmedicine/article?id=10.1371/journal.pmed.1002701, licensed under CC-BY 4.0
Within our hospital system, patients who are at high risk for postoperative complications are referred to a specific clinic before surgery where a team of experts works with patients to “tune-up” a patient’s health so that the patient’s risk for complications after surgery is minimized. “Tune-ups” can mean starting a medication to lower blood pressure or blood sugars prior to surgery. However, determining who is at risk for postoperative complications is extremely difficult. Our models were built to help physicians determine who should be sent to these specialized preoperative clinics. Through our work, we demonstrate that our models have a higher sensitivity and specificity than expert opinion alone. Paired with physician expertise, these models can identify patients who are at high risk for serious complications, potentially lowering healthcare costs due to the prevention of complications as well as specifically targeting patients who require preoperative intervention.
The only way machine learning can have a real impact in the healthcare field is when it is paired with the healthcare provider to support decisions in a way that is embedded in specific workflows. This partnership is our greatest challenge in incorporating this type of technology into one of the most complex sectors: healthcare. By working together, combining experts in technology and healthcare, we can strive to solve some of healthcare’s biggest problems.
These findings are described in the article entitled Development and validation of machine learning models to identify high-risk surgical patients using automatically curated electronic health record data (Pythia): A retrospective, single-site study, recently published in the journal PLOS Medicine.
Reference:
- Corey KM, Kashyap S, Lorenzi E, Lagoo-Deenadayalan SA, Heller K, Whalen K, et al. (2018)
Development and validation of machine learning models to identify high-risk surgical patients using automatically curated electronic health record data (Pythia): A retrospective, single-site study. PLoS Med 15(11): e1002701. https://journals.plos.org/plosmedicine/article?id=10.1371/journal.pmed.1002701