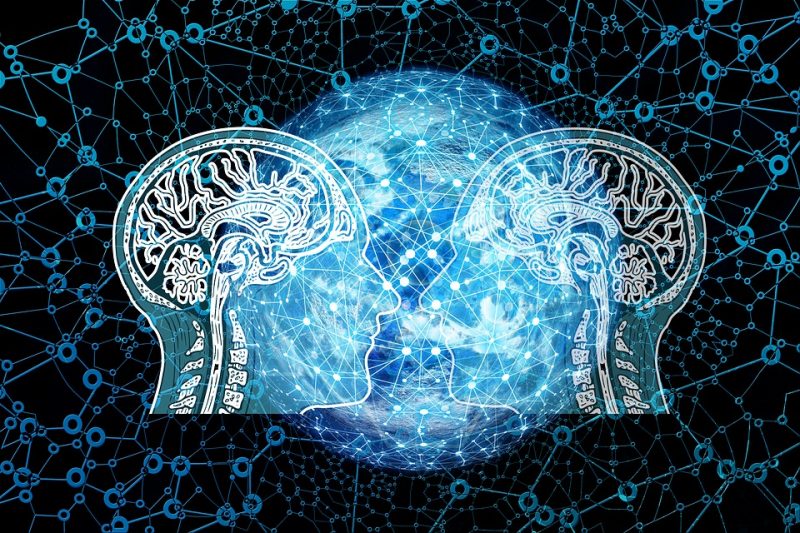
Published by Xin Guo
Zhengzhou University
These findings are described in the article entitled Joint Intermodal and Intramodal Correlation Preservation for Semi-paired Learning, recently published in the journal Pattern Recognition (Pattern Recognition 81 (2018) 36-49). This work was conducted by Xin Guo, Song Wang, Yun Tie and Lin Qi from Zhengzhou University, and Ling Guan from Ryerson University.
In the real world, it is common that one object is able to be observed from different views. Such multi-view observation often leads to a better understanding of the object. This ideology has guided our studies that exploring data from multiple views can acquire richer information than that from a single view in machine learning.
Most of the current studies consider the multi-view features as a one-to-one correspondence, which we call a fully-paired situation. However, this fully-paired requirement is difficult to satisfy in practice, due to numerous reasons like the sensors’ frequencies at different views not being synchronized, or due to missing features extracted from certain views. In such situations, methods have been proposed to figure out the semi-paired problem by exploring the relationship between the samples, paired and unpaired, and their neighbors. But only the structure information from individual views is captured in these methods, which limits the level of performance improvements these methods are able to offer.
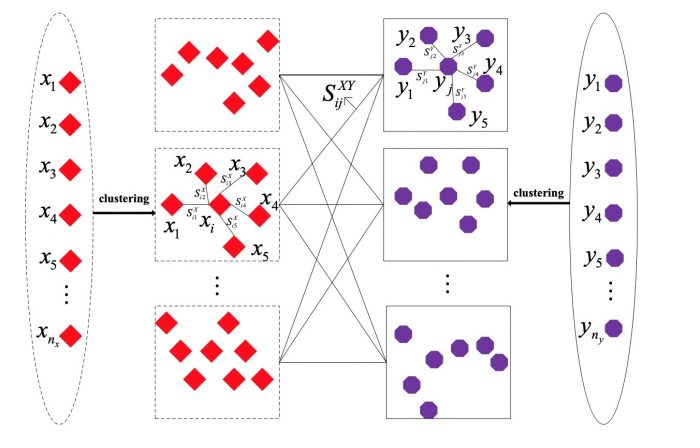
Credit: Xin Guo
To improve learning performance under the semi-paired situation, there are three challenging problems to be addressed urgently, which are: (1) For unpaired multi-view samples, how can we generate the relationship among different views? (2) How do we exploit the discriminative information in the situation when there is no label information available at all? (3) How can we jointly optimize the cross-view correlation and within-view similarity simultaneously during the learning process?
For problem (1), the information of within-view neighborhood relationships and cross-view pairwise samples are used to estimate the cross-view correlation. Intuitively, if a sample from X-view and the other one from Y-view share more co-occurring paired neighbors, there is a higher probability they should be paired. To accelerate the procedure, instead of searching co-occurring paired neighbors from all the sample set, we only select the neighbors that are from the same cluster.
For problem (2), we make a reasonable cluster assumption that the neighboring samples are more likely to be from the same class. Thus, although there is no label information available, discriminative information can be exploited and the similarity within the same class can be preserved.
For problem (3), we combine the within-view correlation and cross-view correlation into a joint optimization problem. Fortunately, the joint optimization problem can be transformed into a typical generalized eigenvalue problem and solved in a close form.
To validate the effectiveness of the work, the proposed methods are compared with several existing related methods on both synthetic data and popular real-world datasets, i.e. UCI multiple feature dataset, UCI internet advertisement dataset, and Wiki dataset. All the experiments demonstrate that the proposed method achieved much better performance than the related methods.