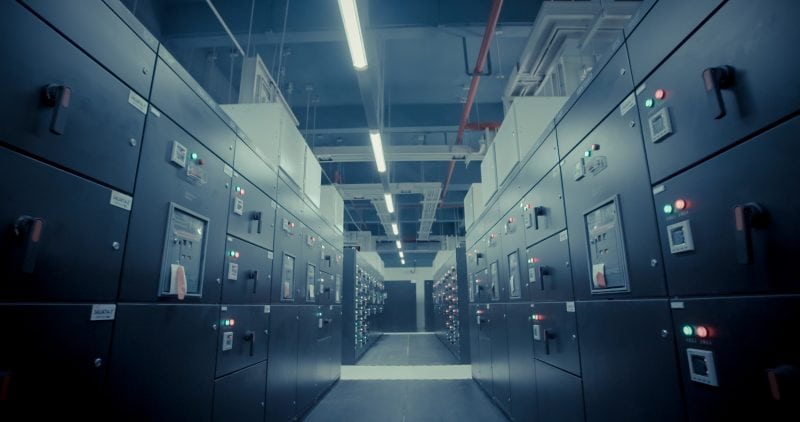
Data Centers mainly function to host the required electronics for computing, telecommunications, and storage. Among other things, they enable the internet as we know it today (streaming, cloud computing), which explains how they proliferated during this decade in accordance to the worldwide internet demand growth, with no apparent deceleration in the foreseeable future.
Consequently, the data centers’ global energy consumption is expected to remain on a steady rise, reaching close to 60 GW of power requirement by 2020 [1] (Figure 1).
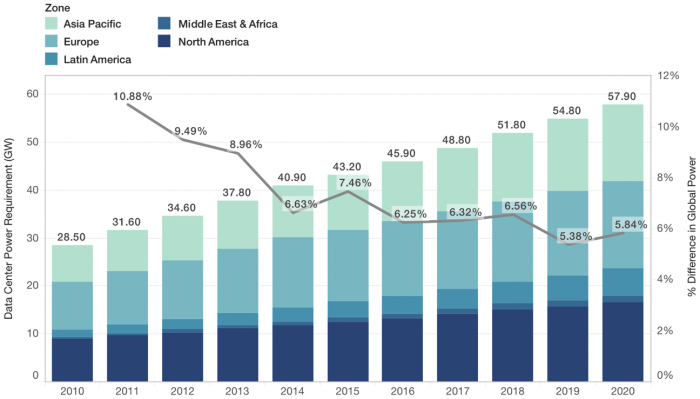
Figure 1. Evolution in worldwide data center energy consumption. (Republished with permission from Elsevier)
Anyone that has experience using computers, even as an occasional user, knows that such devices can reach uncomfortable temperature levels when subjected to demanding tasks (physics simulations, video editing) since the electronics need to dissipate more and more heat to keep functioning. Data Centers are no different as they contain a collection of continuously running computers stacked in proximity to one another. This requirement for high power dissipation explains why cooling represents nearly 50% of the overall energy consumption in Data Centers [2].
Perimeter cooling is a common thermal management practice found in data centers; as shown in Figure 2, it works as follows:
1. A cooling unit delivers cold air into a raised floor that contains perforated tiles through which the airflow enters the cold aisle.
2. The servers suction the cooling flow that removes heat from the electronics. Subsequently, this higher temperature air is delivered into the hot aisle.
3. The warmer air is usually channeled at the ceiling level and returned to the cooling unit to complete the cycle.
One inefficiency that emerges, especially in legacy data centers, is the so-called “thermal short-circuiting” wherein the cooling flow mixes with warmer air leaking from the hot aisle. This implies that the air enters the servers at a higher temperature than it is supposed to, leading to energy waste because of this suboptimal cooling.
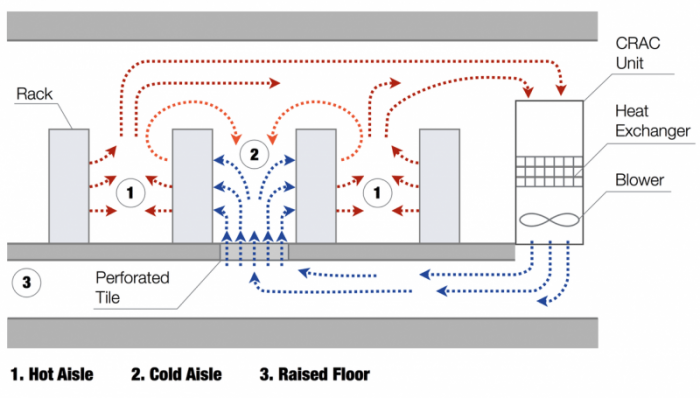
Figure 2. Perimeter cooled data center airflow. (Republished with permission from Elsevier)
Empirical testing in data centers presents some inconveniences:
- The complexity of the physics involved in data center cooling hinders its scalability for laboratory analysis
- Large data centers could become prohibitive for accurate testing in terms of the amount of equipment required and the associated money investment
- Sensors and probes may obstruct the airflow and diminish the cooling performance
Computational Fluid Dynamics (CFD) offers accurate approximations of the data center physics (fluid dynamics, heat transfer) at a fraction of the cost and time when compared to experiments. This is commonly done via commercially available software capable of simulating the data center behavior under basically unlimited scenarios. Thanks to CFD we can make quick predictions that lead to more intelligent decisions when designing and/or diagnosing data center thermal management.
Exergy is a thermodynamic quantity defined as the “available work” in a given system, thus the idea of Exergy Destruction is synonymous with a loss of available work and helps to quantify the system’s inefficiencies. In data center cooling the Exergy can be destroyed mainly for two reasons: (1) the aforementioned “thermal short-circuiting”, and (2) the dissipation of flow momentum due to pressure drop and fluid friction. In this work, we combined the concept of Exergy Destruction with CFD to locate and quantify inefficiencies during cooling air delivery.
Once the Fluid Dynamics and Heat Transfer are properly computed by the CFD tool, we can post-process the Exergy Destruction as follows [3]:
Exergy Destruction due to heat dissipation
Exergy Destruction due to momentum dissipation
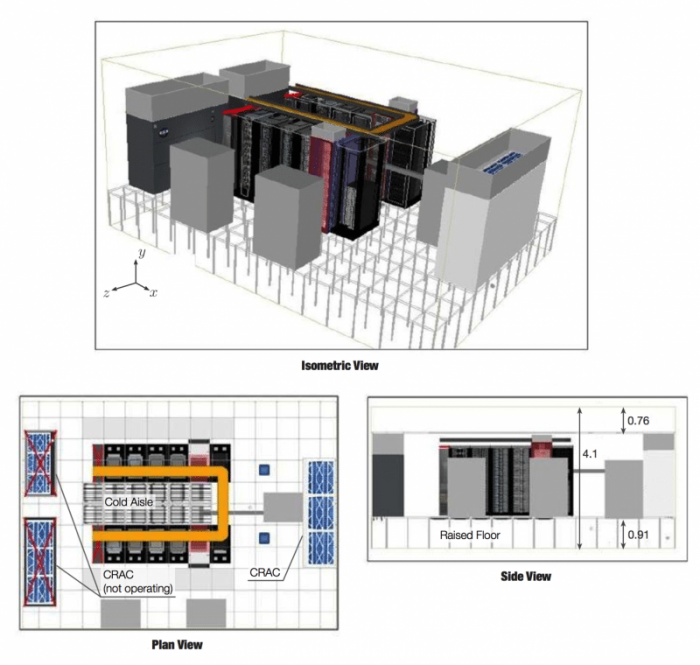
Figure 3. Representation of the data center model used in the numerical simulations. (Republished with permission from Elsevier)
Figure 4 demonstrates the relationship between temperature and pressure with the two modes of Exergy Destruction observed:
1. Thermal short-circuiting: The areas with the most notable temperature gradients (Figure 4 c) encompass the largest Exergy Destruction (Figure 4 a) due to thermal dissipation via heat conduction between warmer and colder airstreams.
2. Pressure drop: Figure 4 b shows that the majority of the Exergy Destruction due to dissipation of momentum exists in the raised floor and the proximity of the perforated tiles, which is where we observed the largest pressure drops (Figure 4 d).
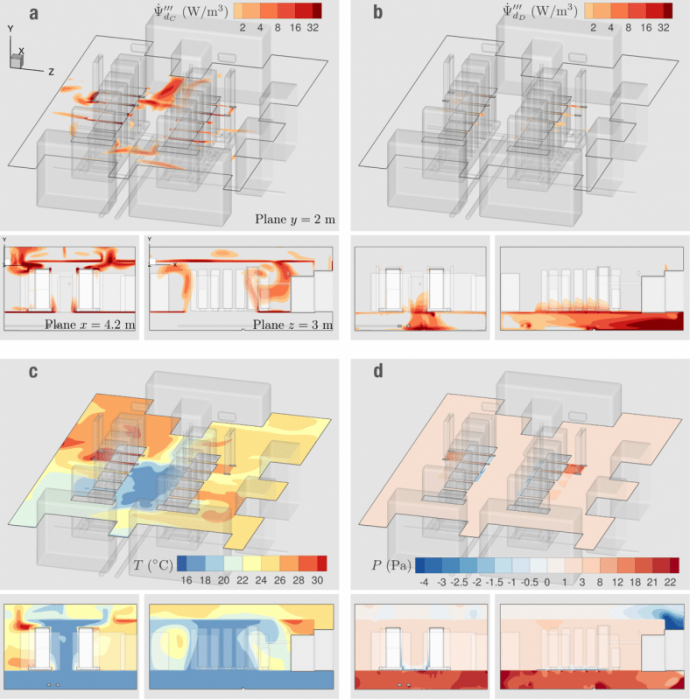
Figure 4. Distribution in the Data Center’s Airside: (a) Exergy Destruction due to heat dissipation, (b) Exergy Destruction due to momentum dissipation, (c) Temperature, (d) Pressure. (Republished with permission from Elsevier)
The Airside Exergy Destruction accounted for an important percentage of the total inefficiencies (Figure 5), demonstrating the importance of its accurate prediction. From this we can improve our decision making toward energy efficient practices.
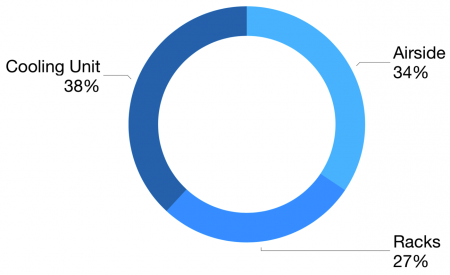
Figure 5. Exergy Destruction associated with each Data Center component. (Republished with permission from Elsevier)
These findings are described in the article entitled Determining wasted energy in the airside of a perimeter-cooled data center via direct computation of the Exergy Destruction, recently published in the journal Applied Energy. This work was conducted by Luis Silva-Llanca from the Universidad de La Serena, Alfonso Ortega from Santa Clara University, Kamran Fouladi from Widener University, Marcelo del Valle from Villanova University, and Vikneshan Sundaralingam from the Internap Corporation. This project was mainly supported by the National Science Foundation; partial findings were sponsored by CONICYT Chile.
References:
- Mattin Grao Txapartegi and Eric Mounier, “Data Center Technologies: New Technologies and Architectures for Efficient Data Centers”, Yole Development Market & Technology Report (2015).
- Van Heddeghem W, Lambert S, Lannoo B, Colle D, Pickavet M, Demeester P. Trends in worldwide ICT electricity consumption from 2007 to 2012. Comput Commun 2014;50:64–76.
- Kock F, Herwig H. Local entropy production in turbulent shear flows: a high-Reynolds number model with wall functions. Int J Heat Mass Transf 2004;47(10):2205–15.