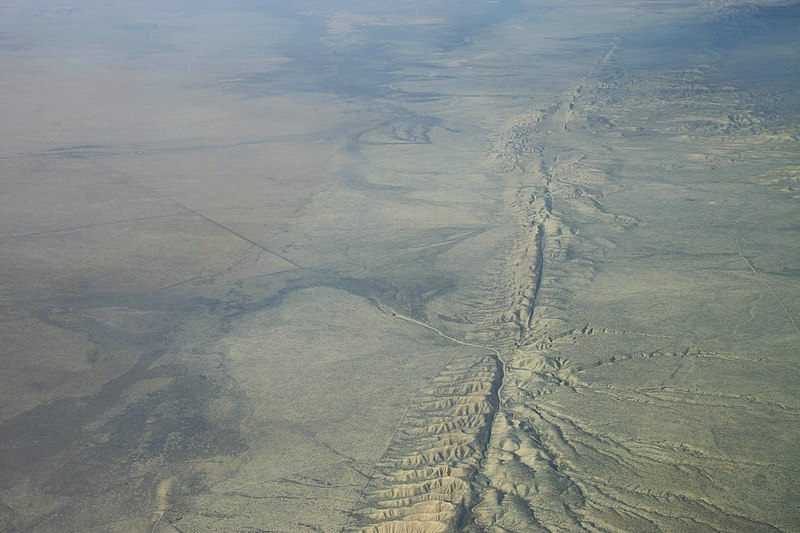
A new tool has begun to emerge in the science of disaster prediction. Seismologists are turning to artificial intelligence to help analyze, understand, and forecast earthquakes.
The Difficulty Of Predicting Earthquakes
Unlike other natural disasters such as hurricanes, tornadoes, and forest fires, earthquakes are extremely difficult to predict. While better mathematical models and superior satellite tracking technology have enabled improved forecasting of weather-related disasters, earthquake prediction has remained spotty at best. Some of the most destructive earthquakes in history such as the 2011 quake in Japan, the Haiti quake in 2010, and the earthquake that struck China in 2008 occurred in regions that were thought to be relatively earthquake safe.
As reported by the New York Times, in order to better understand where earthquakes are likely to form, the conditions that are likely to create earthquakes, and the impacts earthquakes will have on a region, more and more scientists are turning to the power of artificial intelligence and the analysis of big data.
Given how complex and difficult it is to predict earthquakes, as well as the many failures in earthquake prediction that have been witnessed in the past, many scientists are hesitant to even use the word “prediction” when discussing the utilization of AI in earthquake analysis. “Forecast” is the preferred term. That said, the eventual goal of the AI related earthquake analysis is the more accurate forecasting of earthquakes.
Currently, the primary way that earthquake hazards are communicated is through an earthquake probability map. The probability maps show a geographical region with areas highlighted in accordance with their calculated earthquake probability. Probability maps are created based on complex mathematical formulas that must account for many different variables like an area’s distance from a fault, the speed that both sides of the fault line are moving at, and the previous history of earthquakes in that region.
However, these probability maps are just that – maps of probabilities, and the critics of the maps say that the current models used to create them are very general and imprecise, rendering them of limited use in preparing for earthquake disasters. As an example, a map of earthquake probabilities in Southern California might show Los Angeles along with the probability that an earthquake will result in the powerful shaking of buildings within a specified timeframe. A given timeframe for an earthquake map might be 50 years, but in practice the timeframe for earthquake occurrences can be much shorter or longer than that.
One of the more famous studies done on earthquakes in Southern California was conducted by USGS geologist Katherine M. Scharer, and it analyzed the dates of nine previous earthquakes in the San Andreas Fault region. The last major earthquake in the region occurred over 150 years ago in 1857. An analysis of the time span between earthquakes reveals that the average interval between massive quakes was approximately 135 years. From this, it is often concluded that Southern California is due for a massive earthquake any day now. Yet simply taking the average of years between earthquakes isn’t the best method of predicting quakes, because as the study showed the number of years in between big quakes varied wildly from 44 years all the way up to over 300 years.
Improving Forecasting With Artificial Intelligence
As a response to the imprecise nature of current earthquake forecasting models, new models are being based on the analysis of seismic data by neural networks, artificial intelligence systems. According to seismologists like Zachary Ross from the California Institute of Technology’s Seismological Laboratory, as reported by the New York Times, the seismic data that the networks analyze is similar in relevant ways to audio data used to train spoken word processing devices like the digital assistants created by Amazon and Google. The seismic data is passed through a neural network which attempts to find relevant patterns in the massive databases used.
“Rather than a sequence of words, we have a sequence of ground-motion measurements,” said Zachary Ross to the New York Times. “We are looking for the same kinds of patterns in this data.”
The advantage of neural networks and machine learning algorithms is that they can analyze massive amounts of data very quickly, pulling out relevant patterns in an extremely short amount of time compared to the time it would take scientists to do by hand. Brendan Meade, one of the first scientists to utilize machine learning techniques to analyze earthquake data, found that a neural network could complete an analysis approximately 500 times faster than it normally took researchers.
Furthermore, the neural networks didn’t just complete analyses quicker than humans, they also discovered new relevant patterns within the data, leading to new insights that could potentially increase the viability of earthquake forecasts, with Meade’s initial paper demonstrating that the techniques could successfully forecast aftershocks.
Elsewhere, machine learning techniques are being developed by the Los Alamos national laboratory to find new signals which could be relevant in earthquake forecasting. The Los Alamos team found that their system identified sounds made by a fault line as an indication of an impending earthquake, even though this particular sound was regarded as meaningless by previous research. It should be noted that the experiment was done with a simulated fault and not a real one, though hopefully the findings of the team hold true when applied to real faults.
Collecting More Data
The limiting factor here is likely data. More data means better chances to discover viable patterns. As seismic sensor technology improves and sensors become both smaller and cheaper to manufacture, scientists will be able to gather and then analyze much more data. Researchers hope that the techniques will be refined in the coming years and that they can lead to the creation of systems which can more accurately forecast earthquakes, picking up on environmental cues and using them to discern the epicenter of the quake as as well as where and how fast the quake will spread.
There are other methods of collecting information about earthquakes as well. In 2016 researchers from the University of California, Berkeley released an app named MyShake, which uses the accelerometer in smartphones to track how much shaking is happening in any specific area. When the accelerometers detect motion, information is sent to a server that aggregates the data and then analyzed. If many phones with the app detect shaking in an area, the system could detect the epicenter of the event and automatically send out an alert to people in the surrounding area, giving them time to get to cover. Real time data from mobile devices combined with AI analysis could help create early warning systems and assist scientists in understanding how earthquakes manifest in different geographical regions.
Nations like Mexico and Japan have their own earthquake early warning systems, and the state of California has just introduced its own early warning system. These early warning systems may only afford a few seconds of warning in the case of a quake, but those few seconds could be enough for people to get to a safer portion of their building, enough time to get to cover under a safe object. Currently, California’s system is only planned to warn first responders and transit agencies, but in a few years a public warning system is likely to be introduced. Neural networks could play a huge role in the increasing sophistication of these early warning systems.