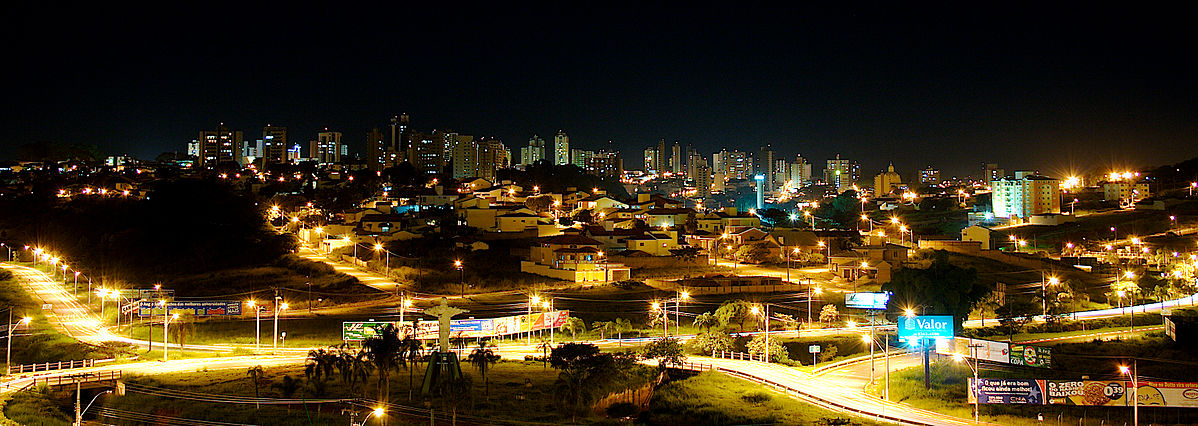
Nowadays, we are continually facing mobility issues caused by the high number of circulating automobiles and people. These issues are more familiar to large cities, such as metropolis and megalopolis, but we can already note locomotion issues to a lesser extent in countryside cities.
Lack of mobility is a problem that usually causes several other sub-problems, such as the lack of street mobility, increasing of prices related to the transportation of people and goods, as well as reducing the efficiency of time-sensitive services. The latter refers to the case of the displacement of ambulances, fire engines, and police-related vehicles. We have a few possibilities to solve (or lessen) such problems, for instance, through the better planning of neighborhoods and cities, allocation of facilities (hospitals, schools, and police stations) in places of easy access to other regions of a city, or even avoiding less access-friendly sites.
For such tasks, computer-based techniques showed promising features in both urban design and street planning. In the last decade, complex networks, which are the basis of our methods, have been used to model real and synthetic systems, from neural connections and power grids to street networks and sewage systems. Complex networks are a powerful toolset to model data focusing on the relations between the entities found in a given data set. These networks, as mathematical models, stand out due to their algebraic properties and computing potential, with analytic applicability to brace cognitive processes of knowledge discovery and decision-making. In the case of cities, the entities of the network are street crossings and the relationships are streets connecting two entities. This way, the resulting network preserves not only the mobility information of cities’ streets but also their geometry and distance.
Through street meshes modeled as complex networks, this research contributes with a toolset to track and reduce intrinsic problems in the urban design of cities caused by the misallocation of points of interest. Such problems are referred to as urban inconsistencies. The toolset tracks inconsistencies regarding displacements of both pedestrians and automobile vehicles. At the same time, it suggests relocations of points of interest to reduce the number of inconsistencies. Our results were validated over the Brazilian city of Sao Carlos, showing how the techniques behave in a real-world example using both quantitative and qualitative analysis.
More specifically, the toolset assumes that street networks should provide the shortest routes among different destinations of the same kind (hospitals, police stations, and schools); when considering a site that is closer to a point of interest by geodesic distance and closer to another by shortest (directed or undirected) path distance, the site is regarded as an inconsistency. To the task of reducing urban inconsistencies, the toolset tracks different sites in the street network that are proper to bear a point of interest. In other words, the algorithm greedily searches for places that reduce rather than eliminate inconsistencies. These places are the output of the algorithm, and they support tasks of urban data-driven decision-making. It is noteworthy mentioning that this process is suitable both for enhancing the location of existing cities’ points of interest and also for planning entire cities and neighborhoods from scratch.
Although we focus on pedestrians and automobiles, the proposal is suitable for any variety of on-surface transportation (e.g., bicycles, and buses, to name a few), as they follow the paths of street networks. On the whole, concerning other domains, our proposal can track routing problems of computer networks, aid the topological design of electronic circuits, enhance the location of facilities in transportation networks, and much more.
These findings are described in the article entitled Detecting multi-scale distance-based inconsistencies in cities through complex-networks, recently published in the Journal of Computational Science.