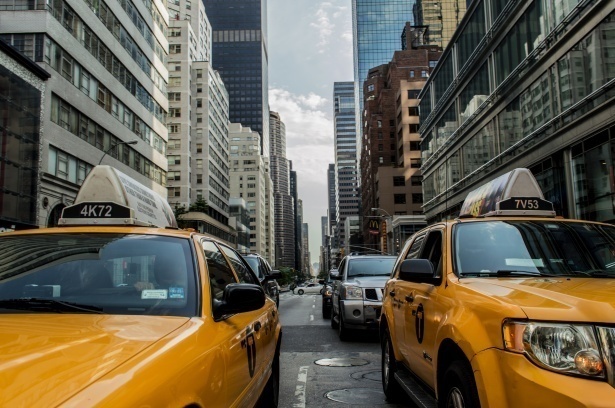
The use of artificial neural networks and other types of prediction models of biological inspiration are used in a huge variety of applications, covering very different areas ranging from neurobiology and psychology to applied engineering. In the world of mechanical engineering (and more precisely, engines), these models have been used to predict noise, octane fuel, and some pollutant gases, among others.
The application of artificial neural networks for the prediction of pollutant gases from engines began in the 90s, but with the improvements in artificial intelligence, and also in algorithms and computer efficiency, in recent years, an important upturn has been observed due to the high capacity of these models to predict variables that due to their behavior are very difficult to predict through more conventional methods as linear regressions.
Predicting the engine exhaust pollutant emissions in a fast and computationally economic way allows to reduce the number of engine test bench experiments, and if the results are optimal, these predictions have a high value since they could allow corrective measures to reduce the pollutant emissions that are highly harmful to health, such as nitrogen oxides or nanoparticles. Among these corrective measures, we can name activation of exhaust gas post-treatment systems (catalysts), activation or deactivation of exhaust gas recirculation or regeneration of the diesel particulate filter.
In this framework, our work was carried out in order to develop, evaluate and compare two predictive models, artificial neural network model and symbolic regression model, of the instantaneous exhaust emissions in transient and steady-state conditions of a diesel 2.0 TDI engine operating with different proportions of animal fat/pure diesel and using as input the variables that can be read by the electronic control unit. Animal fat biodiesel is a second generation biodiesel from waste, favoring the circular economy. In addition, previously, it was shown that this biofuel had some environmental advantages in comparison with conventional diesel [1].
Artificial neural networks are computer programs, inspired in biological models, that simulate how the animal brain processes information. Artificial neural networks develop their knowledge through patterns and relationships between data and can learn from experience, not programming. Any artificial neural network consists of hundreds of artificial neurons, called single units or processing elements, connected with weights, whose values are increased or reduced to make the connection between neurons stronger. Artificial neural networks are organized in layers, from 1 to n, based on the difficulty of the problem. Artificial neurons, weights, and layers build the neural structure [2]. In this work, a fully-connected multilayer perceptron with just a single hidden layer has been used (Figure 1). The model used had 9 inputs, and 5 outputs, 1 layer, and 40 neurons or hidden units.
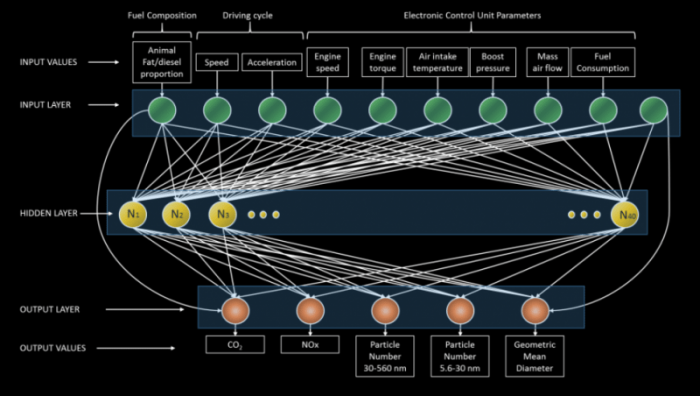
Figure 1: Fully connected feed-forward multi-layer perceptron with one hidden layer. (Credit: Aida Domínguez-Sáez)
Symbolic regression is a specific type of genetic algorithm. Genetic algorithms are based on the idea of the process of natural selection, the best-adapted individuals will have children and will transmit their genes. The symbolic regression is characterized by each individual or candidate solution adopts a tree structure and each of its leaves is called a node. The nodes can be operators or variables. The operator nodes represent a mathematical function while the variable nodes represent the model inputs. Each tree/individual/solution can be reconstructed as a regression function. One of the main advantages of symbolic regression is to provide a final equation that can be used to know the qualitative contribution of each of the input variables.
The application of these models to experimental data obtained in engine test bench, allowed to predict NOx, CO2, numerical concentration of particles in a size- range of 30-560 nm and the geometric mean diameter of the particles in transient conditions, with coefficient of determination (R2) equal to 0.91, 0.78, 0.87, and 0.81, respectively, for artificial neural networks. The prediction by symbolic regression has provided an R2 of 0.91, 0.82, 0.87 and 0.82 for the same pollutants. Both models provided similar prediction results regardless of the fuel used.
The diesel engines, and also the most modern gasoline engines, emit the so-called particles in nucleation mode, these nanoparticles are highly dangerous to health due to their ability to penetrate the deeper reaches of the respiratory tract. This type of particle has a certain randomness in its formation, so the predictive capacity of the models is much more limited than in the case of the rest of the pollutants. The studied models supplied R2 equal to 0.41 and 0.40, for the symbolic regression and the neural artificial networks, respectively.
Both models present similar coefficients of determination, except for the emission of nitrogen oxides (NOx), in which symbolic regression predicts with greater accuracy. One of the most relevant conclusions of this work is the feasibility of the modeling of particle number emission in accumulation mode and size (geometric mean diameter) through two models of biological inspiration, opening the door of the research area of particle number-size distribution predictions of diesel/gasoline engine emissions.
These findings are described in the article entitled Prediction of exhaust emission in transient conditions of a diesel engine fueled with animal fat using Artificial Neural Network and Symbolic Regression, recently published in the journal Energy. This work was conducted by Aida Domínguez–Sáez, Giuseppe A. Rattá, and Carmen C. Barrios from the Research Centre for Energy, Environment and Technology.
References:
- Barrios CC, Domínguez-Sáez A, Martín C, Álvarez P. Effects of animal fat based biodiesel on a TDI diesel engine performance, combustion characteristics and particle number and size distribution emissions. Fuel, 2014:117(Part A):618-623.
- Agatonovic-Kustrin S, Beresford R. Basic concepts of artificial neural network (ANN) modeling and its application in pharmaceutical research. Journal of Pharmaceutical and Biomedical Analysis 2000: 22(5):717-727.