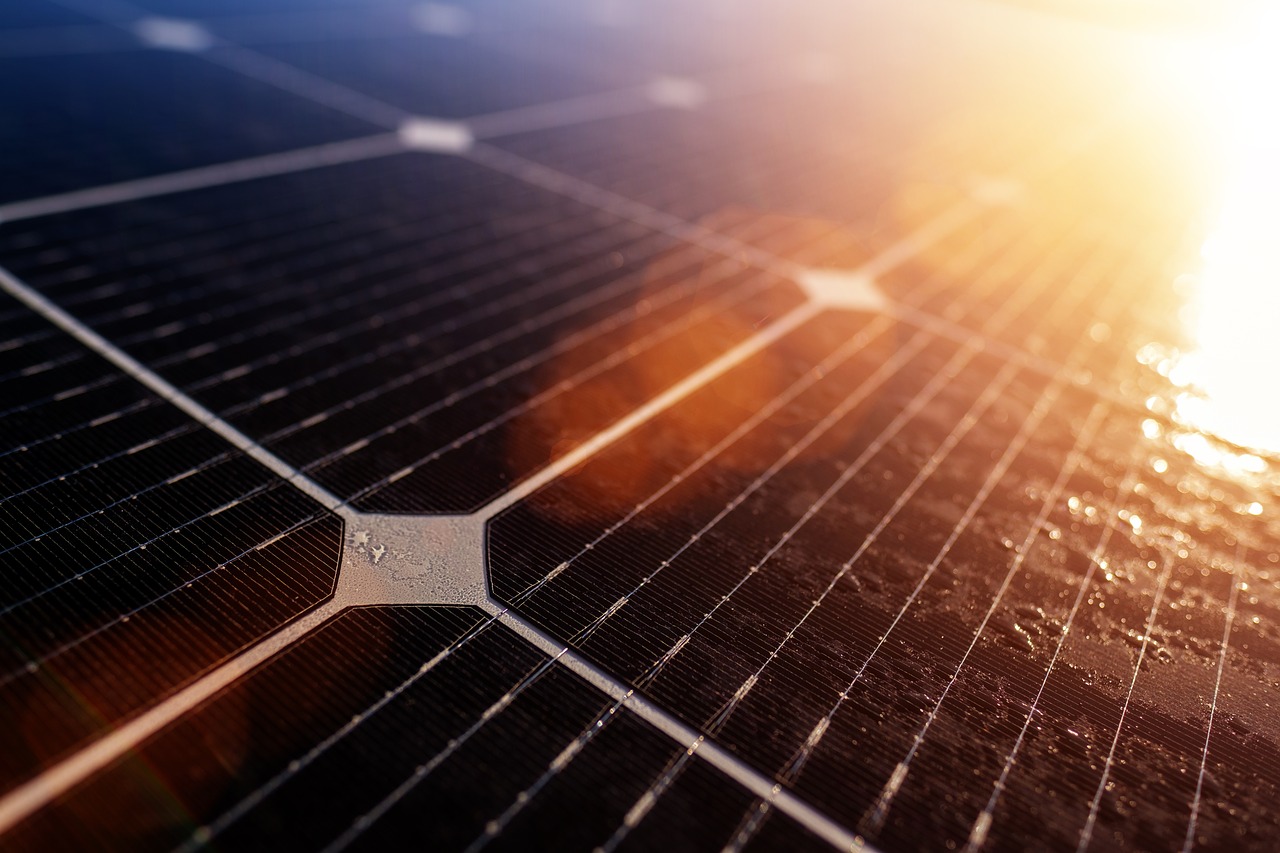
Emergent technologies have been historically predicated on the discovery and development of new materials – silicon computer chips, lithium-ion batteries, gallium nitride LEDs, etc. The space of potential materials that could be made from the more than 80 naturally occurring elements is almost limitless.
To meet the challenges of our growing population while dramatically reducing and ultimately eliminating our reliance on fossil fuels for energy, we need a rational approach for accelerating the discovery of next-generation energy materials. A recent marriage between materials science and machine learning, termed materials informatics, has encouraged great strides towards this goal of accelerated materials discovery, design, and implementation.
Solar cells are a critical area of need for meeting the world’s energy demands with renewable (carbon-free) sources. A class of materials called perovskites have taken this field by storm, with perovskite solar cells nearly doubling in efficiency since their discovery less than 10 years ago.1 The name perovskite comes from Lev Perovski who discovered the mineral, calcium titanate (CaTiO3), in the Ural Mountains almost 200 years ago. Perovskites are an exciting class of materials for many applications because they can accommodate the substitution of many different cations and anions while maintaining the perovskite structure (Figure 1).
The general formula for perovskites is ABX3, where, in the case of calcium titanate, A = calcium (Ca), B = titanium (Ti), and X = oxygen (O). These structures are characterized by a large A-site cation surrounded by a corner-sharing network of BX6 octahedra and are notable for their stability and charge-transport capabilities. In addition to solar cells, these materials have found use as catalysts, electrolytes, and LEDs, among other applications.
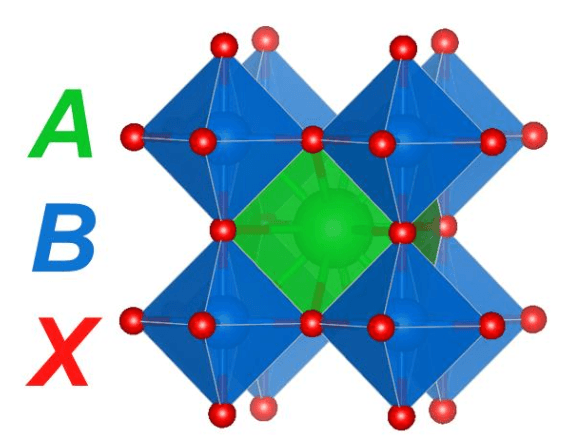
Figure 1. Cubic perovskite structure where the A-site cation is surrounded by a corner-sharing network of BX6 octahedra. Figure courtesy Christopher Bartel.
The most critical property when considering a new material for any application is its stability. If the material is unstable, it will be difficult to synthesize and unlikely to maintain its efficiency when implemented as a device. For example, solar cells are expected to maintain their efficiency on the roof of a house for at least 25 years. Historically, the stability of new materials has been assessed by experimental trial-and-error. A solid-state chemist might imagine a new compound and go to the lab and attempt to make it from a set of potential precursor materials and synthesis conditions. While the intuition of a good chemist can lead to exciting discoveries, this is a costly and time-consuming process. When experimental chemists can be guided by successful rules-of-thumb or “descriptors,” this process can be greatly accelerated. In 1921, Viktor Goldschmidt devised his famous “tolerance factor,” which relates the radii of the A, B, and X ions in a given ABX3 formula and helps indicate whether a given compound is likely to be stable in the perovskite structure.2 Whereas the attempted synthesis of one new compound might take weeks or months, a simple descriptor like Goldschmidt’s tolerance factor can be applied for many compounds in a few seconds. Nearly 100 years after its invention, Goldschmidt’s tolerance factor is still widely used to make an initial guess at whether a compound is potentially synthesizable as perovskite.
In our work, we assembled a database of compounds that are known experimentally to be either stable or unstable in the perovskite structure, tested the predictive power of Goldschmidt’s tolerance factor, and used machine learning to greatly improve upon this descriptor. We found that for a set of > 500 materials, Goldschmidt’s tolerance factor correctly predicts the perovskite stability 74% of the time. Using the same inputs (i.e., the ionic radii of A, B, and X), we applied a statistical learning algorithm called SISSO3 to identify a new tolerance factor that correctly predicts the perovskite stability for 92% of the same set of materials. As with Goldschmidt’s parameter, thousands of potential perovskites can be classified as stable or unstable in a matter of seconds, but now with greatly improved predictive accuracy.
The improvement of our tolerance factor compared with Goldschmidt’s can be found primarily in the vast reduction in false positive rate from 51% to 11%. This suggests that an experimental chemist hoping to synthesize a new perovskite would succeed ~9 of 10 times using our tolerance factor compared with only 5 of 10 using the prior state-of-the-art tolerance factor developed by Goldschmidt. We expect our tolerance factor will be the new benchmark descriptor to provide an initial prediction of whether a given compound will or won’t crystallize in the perovskite structure, reducing the time and expense of the materials development pipeline from identification to synthesis to the application as state-of-the-art materials for emergent energy technologies.
These findings are described in the article entitled New tolerance factor to predict the stability of perovskite oxides and halides, recently published in the journal Science Advances.
References:
- https://www.nrel.gov/pv/cell-efficiency.html
- V. M. Goldschmidt, Die gesetze der krystallochemie. Naturwissenschaften 14, 477–485 (1926).
- R. Ouyang, S. Curtarolo, E. Ahmetcik, M. Scheffler, L. M. Ghiringhelli, SISSO: A compressed-sensing method for identifying the best low-dimensional descriptor in an immensity of offered candidates. Phys. Rev. Mater. 2, 083802 (2018).