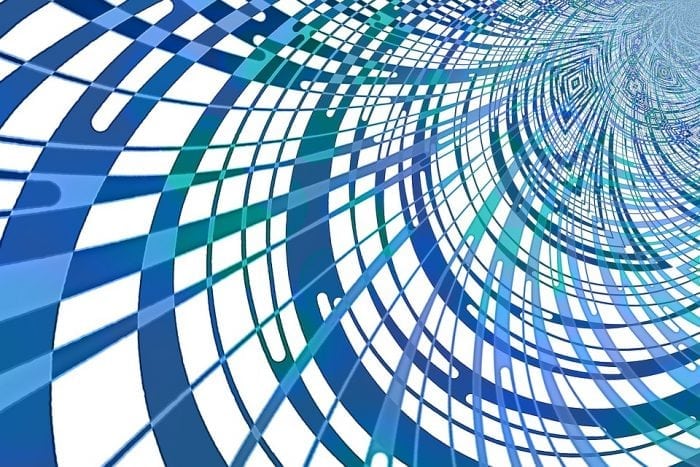
Investigation of the role of the local field potential (LFP) fluctuations in encoding the nervous system’s received sensory information remains largely unknown. On the other hand, the transmission of these translation rules in information transmission between the structure of sensory stimuli and the cortical oscillations to the bio-inspired artificial neural networks operating at the efficiency of the nervous system is still a vague puzzle.
In order to achieve this important goal, computational neuroscience tools can be useful. Therefore, we simulated a large-scale network of excitatory and inhibitory spiking neurons with synaptic connections consisting of AMPA and GABA currents as a model of cortical populations. Based on empirical observations of the learning process in the brain, we developed a spike-based unsupervised weight optimization based on the dynamical behavior of the excitatory (AMPA) and inhibitory (GABA) synapses using Spike Timing Dependent Plasticity (STDP) in identifying MNIST dataset, and we achieved a performance of 96.1%.
Then, we assumed that the information of the trained patterns was stored in the network, which in turn caused the output fluctuations (LFP oscillation) and input patterns, which included shared information. We tested this hypothesis with some steps. First, we computed the LFP and the power spectrum of LFP, and we observed that by applying each digit to the trained network, a peak has appeared at frequencies of 60 to 100 Hz in the LFP power spectrum of a corresponding classifying neuron. Second, we calculated the mutual, joint and redundancy information between the LFP power at frequency f and the input digit. According to the results, we concluded that our assumption was correct, and the input and output of the spiking network were contained shared information; in other words, input pattern information was encoded in the LFP power spectrum.
In the next step, we were looking to propose a more accurate description of the computed quantitative information, which brought us to the topic of Prolate spheroidal wave functions (PSWFs). The interesting result was that the PSWF coefficients of input with the MSE criterion and the output LFP power spectrum with the CIM criterion was equal. The valuable result was that 82.3% of PSWF coefficients of the input digit were the same as the connecting weights of the cortical neurons to the classifying neurons after the completion of the training process. This high percentage of matching between coefficients and synaptic weights can be a gateway to the discovery of the encoding principles of the information transmission in the nervous system.
We introduced the new concept of “cortical spiking model as the information channel transmits input information to the output oscillations in the form of PSWF coefficients.” Given that, the proposed concept has significant application in neuroscience, as well as in the computational capability of spiking network and learning in artificial intelligence; this article is a new horizon following the Hopfieldʼs challenge that will provide intelligence network with the power to compete with the human brain in real-world applications.
These findings are described in the article entitled A new approach to detect the coding rule of the cortical spiking model in the information transmission, recently published in the journal Neural Networks. This work was conducted by Soheila Nazari and Karim Faez from the Amirkabir University of Technology, and Mahyar Janahmadi from the Shahid Beheshti University of Medical Sciences.