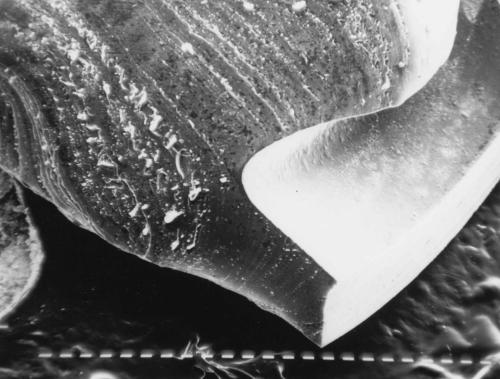
Fatigue represents the loss of a material’s performance (mainly mechanical properties) under constant cyclic, or periodic, loading. In general, researchers only investigate the number of cycles until a failure under constant conditions, which are frequency and applied load amplitude (stress or strain).
Over the years, researchers have proposed several models to predict the number of cycles until failure (N), but the most well-known and simple ones are the S-N or Wöhler curves. However, this analysis can only give information about the conditions under which a sample will most likely fail. This represents an average value over a limited amount of tests due to time constraints, as sometimes millions of cycles are necessary for several samples over a wide range of experimental conditions.
In this case, statistical analysis must be performed, leading to large experimental uncertainty (up to 100%). For design purposes and engineering calculations, large safety factors must be applied to compensate for this high uncertainty in material properties for heavy and larger parts, especially those in automotive, aerospace or petrochemical industries, in order to be on the safe side.
There is a high amount of interest in gaining more precise information about the fatigue behavior of a sample, and the interest is even greater if the measurement can be done online, i.e., to follow some materials’ properties as a function of time or number of cycles rather than reporting only about the ultimate condition: complete failure. The main objective of our work is to propose some methods to relate specific structural changes like crack initiation and propagation, as well as decreasing stiffness and strength, to better predict part failure before it occurs.
All materials can be described as being visco-elastic, and their mechanical behaviors under cyclic loading can be decomposed into a viscous (energy dissipation) and elastic (stored energy) contribution. But the relative contribution of each behavior is very different from one material to another (i.e., a rigid metal vs. a soft rubber).
Since the applied loading (input) is periodic, so is the output. In a periodic signal analysis, the Fourier transform is a powerful tool to extract the most important information: material response. This analysis is now applied on our fatigue tests to detect changes in the material behavior over time.
Our results show that under specific conditions, higher harmonics (even and odd ones from the fundamental one, which is the input) can be detected, and their intensity can give direct information about the fatigue state of a sample. Our analysis showed that variations of these higher harmonics’ intensity (especially the second and third, since they are the most sensitive ones) can now be used as reliable fingerprints to predict material failure and stop a test or application before a catastrophic event like complete part failure occurs.
With this tool, it is expected that more precise characterization can be performed on any material and that lower safety factors can be applied in the future to reduce the cost/weight of critical parts in a system and eliminate possible premature failure by providing warning signals.
These findings are described in the article entitled “Influence of molecular properties on the mechanical fatigue of polystyrene (PS) analyzed via Wöhler curves and Fourier Transform rheology,” recently published in the journal Polymer. This work was conducted by Valerian Hirschberg, Lukas Schwab, Miriam Cziep, Manfred Wilhelm, and Denis Rodrigue from Université Laval and Karlsruhe Institute of Technology.