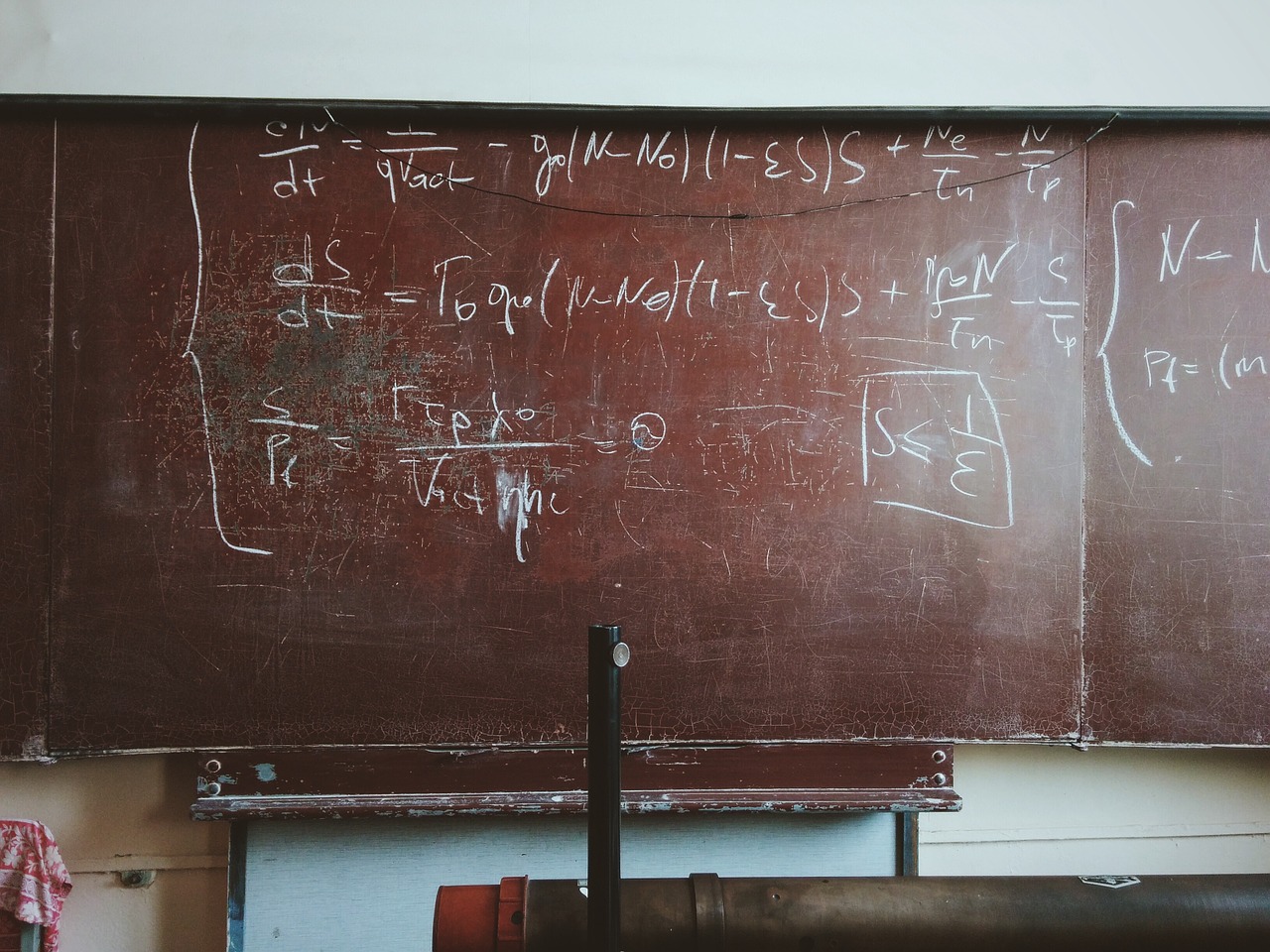
Every one of our bodies contains trillions of cells, each of which plays a specific role to ensure our physiology remains stable, a state called homeostasis. The immune system plays a critical part in maintaining homeostasis by protecting us from the external environment, including everything from disease-causing pathogens to the pollutants we encounter in the air every day. When the immune system faces a challenge, it triggers inflammation, which we recognize by its familiar signs: a fever, rash, tissue redness, or swelling. But what happens when inflammation is present without a good reason?
Under normal conditions, the immune-mediated inflammatory response eliminates pathogens from the body and responds to other inflammatory stimuli, like irritants. However, when the immune system becomes inappropriately activated due to a breakdown in its controls, it stimulates an abnormal inflammatory response, a hallmark of a group of diseases caused by immune system dysfunction, called inflammatory diseases.
This is a broad group and includes neurodegenerative diseases like Alzheimer’s; autoimmune diseases like Lupus and Rheumatoid arthritis; chronic inflammatory diseases like Crohn’s, ulcerative colitis, and endometriosis; and even metabolic diseases like type II diabetes and atherosclerosis. These diseases are complex in that they arise due to an improper immune reaction in the affected organs but have systemic consequences throughout the body. This complexity makes them incredibly difficult to study, which has, in turn, slowed the development of effective treatments.
Inflammatory diseases are not only complex but also highly heterogeneous on a patient-to-patient basis, making an expectation of a one-size-fits-all treatment unrealistic. Currently, treatments for these diseases are focused on alleviating symptoms rather than targeting the cause: disruption of immune homeostasis. These challenges underlie the unacceptably low treatment success rates for inflammatory diseases, which are often debilitating and are amongst the few diseases increasing in incidence worldwide.
Why Are Inflammatory Diseases So Difficult To Study?
The immune system is made up of a multitude of diverse cell types that interact and communicate with each other and surrounding tissues via hundreds of secreted small molecules, like microscopic messenger services. These immune cells and the sum of their interactions are collectively referred to as the immune network, or system. Each individual’s immune system evolves throughout their lifetime in response to everything they encounter in the environment. Thus, the immune systems of any two people are as different as the two individuals themselves.
This is one of the major roadblocks that hinders the development of new therapies for inflammatory diseases. To first approach treating a disease, scientists typically look for biomarkers, single molecule differences between normal tissue and diseased tissue. A targeted therapy against a disease biomarker spares normal tissue (which lacks the biomarker), minimizing side effects. For example, in cancer, scientists have identified biomarkers that are displayed by cancer cells but not by normal cells. A treatment designed to kill cells displaying this biomarker will be selective for the cancer cells because normal cells lack that biomarker.
This approach has worked well for diseases that are driven by such obvious differences between normal and diseased tissue. However, it falls apart when applied to inflammatory diseases because of the heterogeneity between individuals; it is extremely difficult to determine whether immune system differences are due to disease or the normal differences that inherently exist between individuals.
Additionally, approaches to treating inflammatory disease face challenges that stem from the lack of a good system in which to study them. Because we cannot experiment directly on humans, scientists often use mice engineered to have the same molecular disease drivers found in humans, generating a mouse model of the disease of interest. Using these mouse models of human disease, scientists can begin to understand how disruption of a tissue causes disease and can test different therapies in development to determine whether they have effects on alleviating disease.
However, although they are convenient and generally easy to work with, there are many drawbacks to using animals to model human disease. Rather obviously, mice are not humans, and unfortunately for the immune field, the immune systems of mice are wired quite differently than in humans. Because of this, the immune reaction of a mouse may not be the same as a human and therefore, is not the best system in which to study the human response. Given the poor understanding of how inflammatory diseases arise, and the somewhat distinct properties of murine and human immune systems, it is perhaps not surprising that animal models have failed to accurately represent the human condition, preventing them from being as effective as they are for modeling other diseases like cancer.
What Is Systems Biology?
The goal of any disease treatment is to reverse the disease state back to a healthy state. To do this, one must first understand how these two states are different. Given the complex heterogeneous nature of inflammatory diseases, scientists have been forced to develop new ways to understand and study them, turning to math to aid in obtaining increased insight.
Systems biology is a result of new, innovative ways to think about biology using math to comprehend multiple interacting components and processes in a way not feasible for unaided human thought. Generally, systems biology is the computational analysis and mathematical modeling of a biological system with emphasis on enlarging the breadth of perspective on the question of interest (in contrast to reducing it to the more limited scope that would be required in the absence of mathematical frameworks). It maps and explores relationships between biological entities (DNA, RNA, proteins) to form a comprehensive picture of a system that includes all of its dynamic and interactive parts.
Systems biology employs a number of approaches to aid in overcoming the issues presented by a lack of good model organisms and high patient-to-patient variability. In vivo systems biology always begins by sampling the disease. This is done by directly sampling the affected tissue via biopsy or by collecting immune cells circulating in a patients’ blood. The former gives us direct information about the disease, whereas the latter provides information about its systemic features. Although a biopsy is preferable, it is not feasible in all tissues and is always more invasive than a blood draw, which is a standard part of almost every physical exam.
Next, biologists can measure all the biological entities (DNA, RNA, protein) in these samples both at baseline and in response to treatments. Advanced mathematical and computational algorithms are then employed to model the aberrant immune networks and predict features of the disease state. These mathematical approaches include complex artificial intelligence and machine learning.
Importantly, systems biology can integrate the complexity and heterogeneity of inflammatory disease in a holistic manner, without relying on previously defined parameters, and has the ability to incorporate both biological data and clinical information from patients. The information gained using this analysis can then be used to identify diagnostic biomarkers or treatment targets for a given disease.
How Can Systems Biology Inform Better Therapeutic Development?
With inflammatory diseases on the rise and current treatments having disappointing results, it is crucial to explore new ways of understanding these diseases in order to develop new treatments. Systems biology is a key tool in this endeavor. It allows scientists to look for complex patterns of disease features that would be very difficult for a single scientist to find using simple statistics. Using systems biology, we can measure hundreds of disease features from many patients and determine how/if any are associated with different patient subgroups that present with a particular symptom or clinical manifestation. This enables researchers to stratify patients in a more meaningful way. For example, it may help predict which patients will respond to a certain therapy or identify a certain disease feature against which a drug can be designed.
Researchers are currently using systems biology to help propel a deeper understanding of endometriosis, an inflammatory condition of the female reproductive system that affects 1 in 10 women and has profound effects on their quality of life and ability to have children. This disease has many different manifestations and affects the female body in different ways. Unfortunately, it is unknown why the disease arises or what determines which symptoms will affect each individual. Other than exploratory surgery, there are currently no lab tests to determine whether a patient has endometriosis. The Griffith lab at MIT has been working to develop assays to detect endometriosis, as well as to determine molecular signatures of infertility. Their recent study identified particular branches of the immune system that are involved in certain aspects of endometriosis, pointing scientists in the direction of symptom-specific treatments.
Because we do not understand how or why the immune system becomes disrupted, current approaches to treating inflammatory diseases often rely on immune suppression, which can have dangerous side effects due to reduced immunity. Using the mathematical modeling of systems biology, the diseased immune network can be mapped and compared with the healthy network, opening therapeutic doors that were previously closed. Using these comparisons, scientists can pinpoint and target more precise nodes where the immune system is disrupted. This enables inhibition of only the abnormal part of the immune system, allowing the rest of the immune system to behave normally and provide protection.
Systems biology allows us the unique opportunity to study disease in actual patients, rather than relying on animal models that fail to mimic the human condition. Using this mathematical approach, we can sort through huge amounts of data to interpret biological complexity and inform the design of new drugs. Instead of broad-spectrum immunosuppressive drugs with scary side effects, we will be able to tailor drugs to specific patient subgroups and to isolated parts of the immune system, improving treatment efficacy and the patient experience.
These findings are described in the article entitled In vivo systems biology approaches to chronic immune/inflammatory pathophysiology, recently published in the journal Current Opinion in Biotechnology. This work was conducted by Alina Starchenko and Douglas A Lauffenburger from the Massachusetts Institute of Technology.