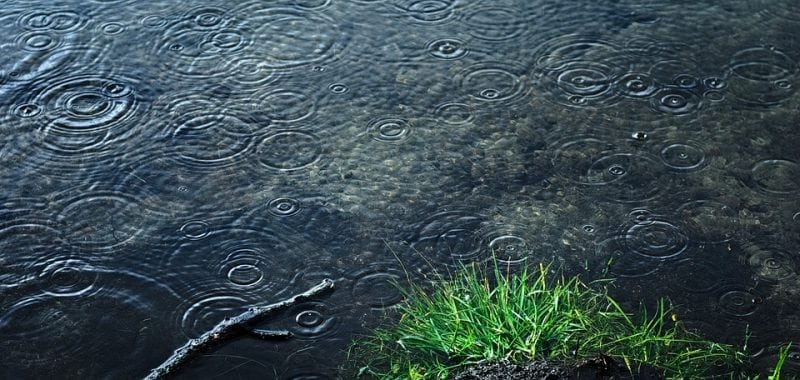
The hydrologic application of precipitation estimation from remote sensing is a promising way to assess streamflow variability and its downstream impact for ungauged basins. Compared to conventional ground-based observational networks (i.e., rain gauge, meteorology station, weather radar), satellite remote sensing is uninhibited by terrain and can provide consistent precipitation measurement at fine space-time resolution (as fine as 0.04°/half-hourly). This is particularly meaningful for catchments characterized by complex terrain that are often susceptible to hydrometeorological hazards (e.g., flood, debris flow, landslides) induced by heavy precipitation and its interaction with catchment geomorphic characteristics.
Current satellite precipitation retrieval algorithms are based on the high-spatiotemporal-resolution observations in the visible–infrared (VIS–IR) spectrum from geostationary (GEO) satellites and the active and passive microwave (MW) sensors deployed on low-Earth-orbiting (LEO) satellites. The VIS–IR techniques have high sampling frequency (15-min/3–4-km, 1-km VIS) but retrieve precipitation indirectly based on cloud-top brightness temperatures. On the other hand, PMW techniques physically link the signal received by the satellite sensors to the size and phase of the hydrometeors present within the observed atmospheric column, but uncertainties arise from their low observational frequency and large sensor field-of-view areas.
It is deemed that precipitation retrievals from either VIS–IR or MW, or the combination of both sensors, suffers from noticeable errors compared to ground-based measurements. A common practice to improve the consistency of remote sensing-based product to ground-based measurements is to merge these two sources. But this is limited by the available number of reliable ground-based networks, which are scarce in some regions of the globe. Thus, to ensure meaningful hydrologic simulations by incorporating satellite remote sensing precipitation with the hydrologic model, it is necessary to understand the products’ error structure and their propagation characteristics in hydrologic simulations.
We set up a hydrologic model for three nested catchments (Swift, Fishing and Tar) of the Tar river basin in North Carolina, USA, with a drainage area of 426 km2, 1374 km2 and 2406 km2, respectively. 160 rainfall-runoff events over the catchments from 2003–2010 were selected as the simulation targets. Eleven quasi-global satellite precipitation products were evaluated with respect to a radar- and gauge-based product in terms of their accuracy in capturing the intensity of event rainfall and runoff.
The eleven satellite precipitation products come from four algorithms. The first two products are from the Tropical Rainfall Measuring Mission Multi-satellite Precipitation Analysis (TMPA). A near-real-time (Trt) and a gauge-adjusted version (Tg) of TMPA were used in the study. These 3-hourly products are at 0.25 °spatial resolution.
The Precipitation Estimation from Remotely Sensed Information using Artificial Neural Networks (PERSIANN) product is another near-real-time product (Prt) considered in the study. A high-resolution version (HPrt) and a gauge-adjusted version of the near-real-time PERSIANN (Pg) were also considered. Prt and Pg are in 0.25°/3-hourly resolution, while the HPrt is 0.04°/hourly.
Also used were the National Oceanic and Atmospheric Administration Climate Prediction Center’s morphing technique (CMORPH) product available at resolutions of 0.25°/3-hourly and 0.072°/half-hourly, abbreviated as Crt and HCrt, respectively. Gauge-corrected versions of these two products, denoted as Cg and HCg, were also included.
Finally, we included the Global Satellite Mapping of Precipitation’s (GSMaP) version 5 Microwave-IR Combined product for our analysis. Similarly, a near-real-time version (Grt) and its gauge-adjusted counterpart (Gg), were used. The two products are hourly and at 0.1° resolution.
The distributions of mean relative error in event rainfall intensity for all events are demonstrated by boxplot in Figure 1. The figure shows that most of the satellite precipitation products, especially the near-real-time ones, tend to underestimate the rainfall intensity. The gauge-adjusted and higher resolution counterparts show medians of error much closer to zero for all the basins. Product-wise, the high-resolution gauge-adjusted CMORPH outperforms the others.
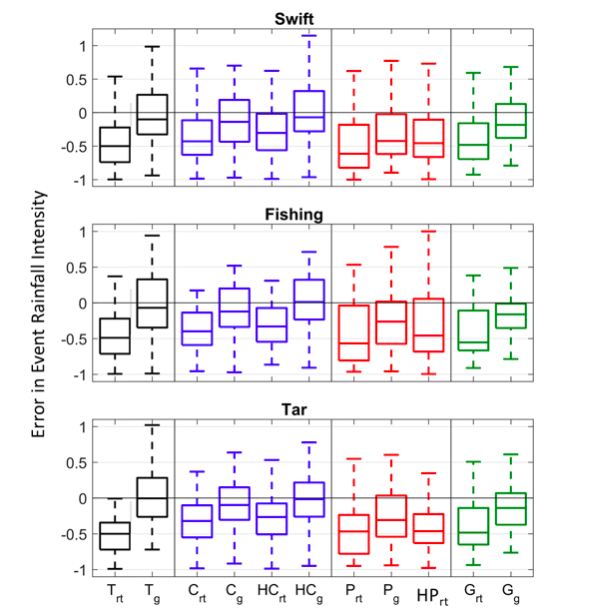
Figure 1. Error in rainfall intensity for all satellite precipitation products over the three study catchments. (Republished with permission)
Mean relative error in event runoff intensity for all events are shown in Figure 2. The satellite product–driven flow simulations show underestimation as to the intensity. Similarly, the gauge-adjusted and high-resolution products are characterized by medians closer to zero than the corresponding near-real-time ones. The medians of error derived from the gauge-adjusted TMPA and high-resolution CMORPH outperform the others in most of the occasions.
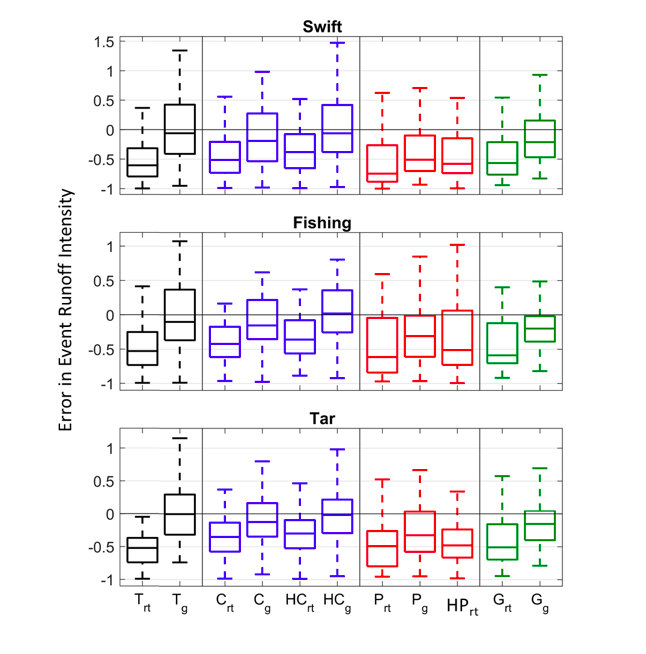
Figure 2. Same as in Figure 1, but for error in event runoff intensity. (Republished with permission)
To sum up, we found that the satellite precipitation products underestimated the intensity of rainfall and runoff of the events examined in this study. The gauge-adjusted and high-resolution products yielded lower error magnitudes than the corresponding near-real-time and coarse resolution counterparts. This indicates the benefit of higher resolution and the usefulness of merging with ground-based observations.
These findings are described in the article entitled Decomposing the satellite precipitation error propagation through the rainfall-runoff processes, recently published in the journal Advances in Water Resources. This work was led by Yiwen Mei, Emmanouil N. Anagnostou, Xinyi Shen and Efthymios I. Nikolopoulos from the University of Connecticut.