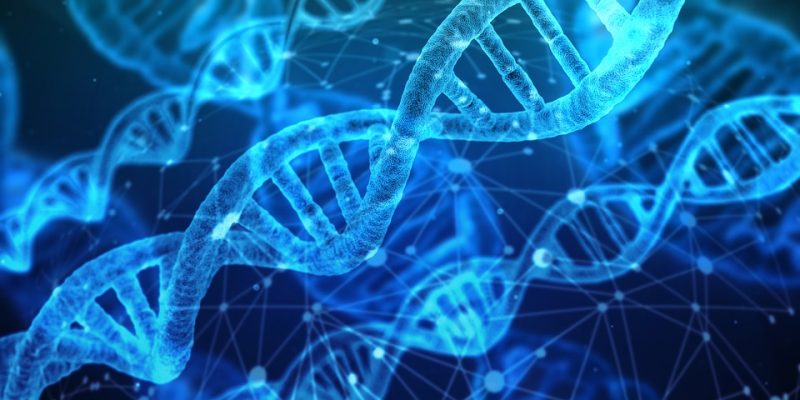
Human disease can be loosely categorized into two broad categories, monogenic and polygenic diseases. The word monogenic means mutating a single gene is sufficient to cause a disease. Cystic fibrosis and Huntington’s disease are some examples that fall into this category. On the other hand, polygenic means that several genes (sometimes hundreds of genes) can influence the disease risk, and each gene only contributes a tiny fraction.
It is relatively straightforward to find the causal gene for monogenic diseases. To do so, scientists collect genetic data from a patient cohort and find the mutations common to all of them. On the other hand, it is much more complex to find the causal genes for polygenic diseases. Each patient may have a different subset of mutations and their mutations can be non-overlapping.
To map the genetic mutations that influence polygenic disorders, scientists have used a technique called genome-wide association studies (GWAS). To understand what a GWAS does, imagine we have two groups of people. Individuals in the case group are sick with a certain type of disease, whereas individuals in the control group are healthy. Suppose for a given genetic locus, we observe that a mutation (colored in red) in case individuals are far more frequent than control individuals, and we can argue that this mutation likely contributes to the risk of this disease.
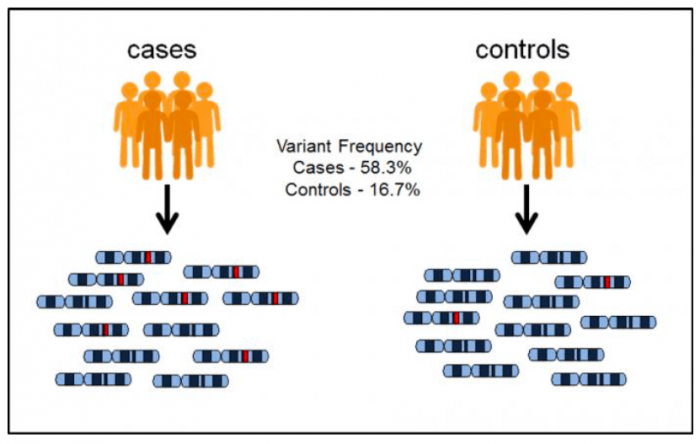
Image source: https://www.ebi.ac.uk/training/online/course/gwas-catalog-exploring-snp-trait-associations-2019/what-gwas-catalog. License: creative commons attribution-sharealike 4.0
During the past decade, GWAS have cataloged an ever-increasing set of disease-relevant variants, from two in 2005 to more than 70,000 in 2018. The majority of these variants (~90%) lie in non-coding regions, or the region that does not code for protein. Although not directly coding for protein, these variants are able to modulate levels of gene expression via regulatory mechanisms, such as via interaction with transcription factors and histone binding proteins.
Unlike variants in the coding region, however, we cannot directly infer the target gene of non-coding variants or the direction of regulation from genetic sequences alone. Because of this, our understanding of the mechanisms through which non-coding variants contribute to disease is limited, despite association signals provided by GWAS. Further, unlike coding variants, where a deterministic relationship exists between genetic variation and the resultant protein sequence, the regulatory functions of non-coding variants are largely contextualized on tissue/cell type as well as the extra-cellular environment. For this reason, disease-relevant cell types and culture conditions must be used in order to maximize power to detect causal relationship while minimizing false discoveries.
To determine target genes of a GWAS variant, scientists use another technique called expression quantitative trait loci (eQTL) studies. Just like GWAS, eQTL studies infer the relationship between genetic mutations and gene expression. One can collect the genotype and gene expression data for a number of individuals, and find the association between genotype and gene expression.
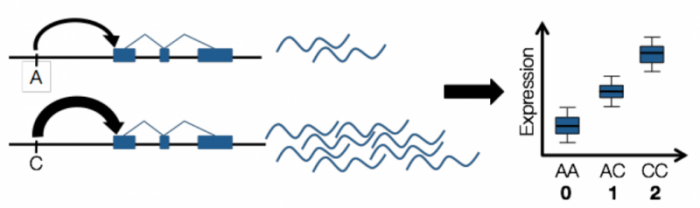
Source: Emily Tsang. License: all rights reserved.
Many research teams have made their eQTL datasets publicly available. One of the largest datasets is from the Genotype-Tissue Expression (GTEx) project, which mapped genetic association with gene expression across 44 tissues (GTEx Consortium et al., 2017). However, the GTEx project mostly sampled tissues (e.g. heart and lung) and does not provide cell-type-specific information. For some diseases, tissue level information is sufficient, but for most other disorders, cell-type level information is needed. Take coronary artery disease (CAD) for example, there exist multiple cell types in the coronary artery, such as immune cells, smooth muscle cells, and endothelial cells. Neither GTEx nor any other publicly available dataset distinguishes these cell types.
To understand unexplained GWAS variants in CAD, Liu and colleagues at Stanford University focused on human coronary artery smooth muscle cells (HCASMC), which has been recently reported to account for 50% of atherosclerotic plaque(Shankman et al., 2015). Genome-wide association studies (GWAS) have identified more than 95 independent loci that influence CAD risk, most of which reside in non-coding regions of the genome. To interpret these loci, Liu and colleagues generated transcriptome and whole-genome datasets using HCASMC from 52 unrelated donors, as well as epigenomic datasets using ATAC-seq on a subset of 8 donors. Through systematic comparison with publicly available datasets from GTEx and ENCODE projects, they identified transcriptomic, epigenetic, and genetic regulatory mechanisms specific to HCASMC.
By jointly modeling eQTL and GWAS datasets, they identified five genes (SIPA1, TCF21, SMAD3, FES, and PDGFRA) that may modulate CAD risk through HCASMC, all of which have relevant functional roles in vascular remodeling. Comparison with GTEx data suggests that SIPA1 and PDGFRA influence CAD risk predominantly through HCASMC, while other annotated genes may have multiple cell and tissue targets. Together, these results provide tissue-specific and mechanistic insights into the regulation of a critical vascular cell type associated with CAD in human populations. In addition, the results from this study provide new leads for downstream experimental validation and a guide for future drug design.
These findings are described in the article entitled Genetic Regulatory Mechanisms of Smooth Muscle Cells Map to Coronary Artery Disease Risk Loci, recently published in the American Journal of Human Genetics. This work was conducted by Boxiang Liu, Milos Pjanic, Ting Wang, Trieu Nguyen, Michael Gloudemans, Abhiram Rao, Victor G. Castano, Erik Ingelsson, Stephen B. Montgomery, and Thomas Quertermous from Stanford, Sylvia Nurnberg, Daniel J. Rader, and Susannah Elwyn from the University of Pennsylvania, and Clint L. Miller from the University of Virginia.
References:
- GTEx Consortium, analysts, L., (LDACC):, D.A.a.C.C.L., management, N.p., collection, B., Pathology, group, e.m.w., Battle, A., Brown, C.D., Engelhardt, B.E., et al. (2017). Genetic effects on gene expression across human tissues. Nature 550, 204-213.
- Liu, B., Pjanic, M., Wang, T., Nguyen, T., Gloudemans, M., Rao, A., Castano, V.G., Nurnberg, S., Rader, D.J., Elwyn, S., et al. (2018). Genetic Regulatory Mechanisms of Smooth Muscle Cells Map to Coronary Artery Disease Risk Loci. Am J Hum Genet 103, 377-388.
- Shankman, L.S., Gomez, D., Cherepanova, O.A., Salmon, M., Alencar, G.F., Haskins, R.M., Swiatlowska, P., Newman, A.A.C., Greene, E.S., Straub, A.C., et al. (2015). KLF4 Dependent Phenotypic Modulation of SMCs Plays a Key Role in Atherosclerotic Plaque Pathogenesis. Nature Medicine 21, 628-637.