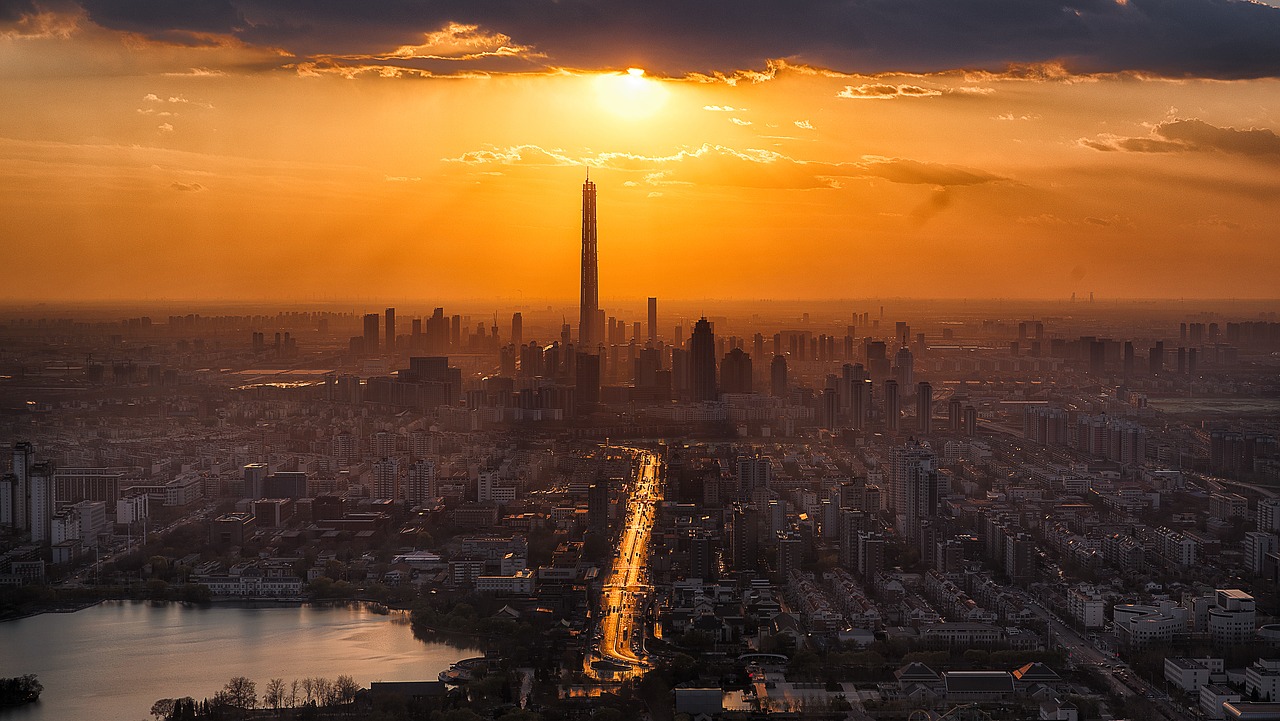
Buildings take a central place in human life. They are globally ubiquitous and are significant consumers of energy. In 2010, the building sector accounted for 19% of all global greenhouse gas (GHG) emissions and 32% of global final energy use, according to the IPCC.
Research shows that for conventional buildings, the operational phase accounts for up to 90% of the life cycle energy demand, with thermal end uses (space heating, space cooling, water heating) dominating the overall demand. According to the IPCC, space heating was the most significant end use in 2010, accounting for one-third of the global building stock’s final energy demand.
Applying the concept of Cyber-Physical Systems (CPS) is a promising strategy to reduce the lion’s share of building energy demand that is complementary to traditional means of building modernization, such as refurbishments.
“Smart buildings as Cyber-Physical Systems: Data-driven predictive control strategies for energy efficiency” illustrates the typical stack of methods from data acquisition and data preparation to building automation system integration and optimization. Moreover, it touches on related areas, such as Multi-Agent Systems, Building Information Models (digital models building design and materials), weather normalization of energy demand, and possible interactions with the Smart Grid. However, at its core, the paper surveys recent research works that use data-driven methods to optimize building service systems’ operation.
For that, it provides an overview of 22 representative works and categorizes them according to the computational methods used, key performance indicators, input data considered, building type studied, impact achieved, and the means of verification. The survey indicates that:
- The majority of the surveyed studies focus on optimizing operational energy costs or energy demand. Most studies take into account thermal comfort, often by formulating a multi-objective optimization.
- Data-driven predictive control studies reach similar effect sizes as studies applying concepts of the field of Model-Predictive Control approaches based on physical simulations of buildings. Neural Networks, and to a lesser extent Reinforcement Learning, are commonly used in the literature.
- For the surveyed works, it is evident that nature-inspired optimization heuristics, such as the Genetic Algorithm or Particle Swarm Optimization, are frequently encountered. Optimization techniques that are robust to perturbations and noise are not prominent in the field of data-driven predictive building control.
- Most works perform validation in the form of simulation. Fewer studies validate their control via experiments, while a minority of studies address the aspect of BMS integration at all. As a consequence, a minority of studies is suitable for integration with routine building operation without additional considerable efforts.
- Few works account for weather conditions when assessing energy performance. While that is appropriate for studies relying on simulations of building physics as conditions are reproducible. However, real-world experiments’ results may be influenced by changing weather conditions. Even fewer works apply statistical methods to draw robust conclusions by quantifying the confidence intervals of the savings they achieve.
- Although the field of predictive building control is related to the CPS concept, none of the works explicitly transfers and applies the findings of the CPS research field.
The overview of state-of-the-art advances in data-driven optimization of building system operation, as well as important related fields, motivates seven research questions. Each intends to guide future research work, as e.g., pursued in my Ph. D. thesis “EVOX-CPS: A Methodology for Data-Driven Optimization of Building Operation”.
These findings are described in the article entitled Smart buildings as Cyber-Physical Systems: Data-driven predictive control strategies for energy efficiency, recently published in the journal Renewable and Sustainable Energy Reviews. This work was conducted by Mischa Schmidt from NEC Laboratories Europe and the Luleå University of Technology, and Christer Åhlund from the Luleå University of Technology.