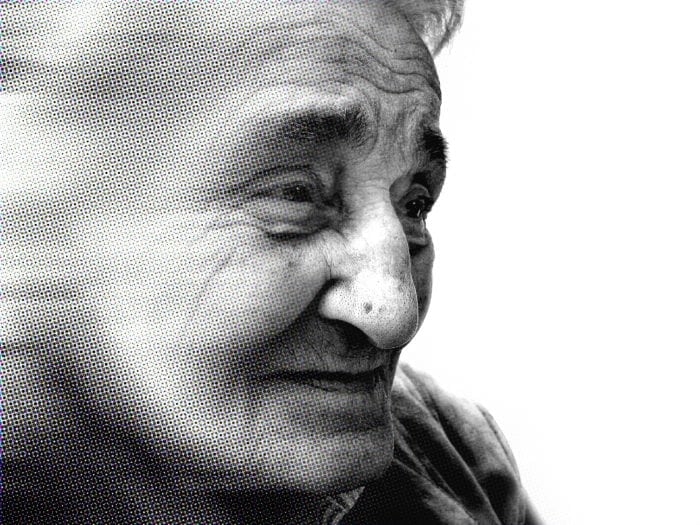
Recent statistics show that about half of the population in the US suffers from at least one type of health problem including mental illness, substance use disorders, or chronic medical conditions (Walker & Druss, 2017). Substance use and mental disorders are both considered brain diseases (Volkow, Koob, & McLellan, 2016).
Brain disorders are a major worldwide problem as also pointed out in studies analyzing data from the World Health Organization Global Survey (Murray & Lopez, 1997; Silberner, 2017). A better understanding of these diseases including techniques for diagnosis and treatment is needed to impact and improve global life expectancy (Kontis et al., 2017).
Intensive research has been performed to study Alzheimer disease (Alzheimer’s, 2012), traumatic brain injury (Zaloshnja, Miller, Langlois, & Selassie, 2008), Parkinson’s disease (Kowal, Dall, Chakrabarti, Storm, & Jain, 2013), schizophrenia (Jablensky, 2000), Huntington’s disease (Ross et al., 2014) and addiction (Volkow et al., 2016). Research suggests that brain disease changes both brain wiring and function. Brain connections can not only be damaged but can also be “re-wired” as a compensatory mechanism (Caroni, 2017).
The fact that some humans can live with only about half of their brain (Vining et al., 1997) provides a good example of this adaptability. There is great interest in disease-related changes. The configuration of brain connectivity has the potential to be an indicator (or biomarker) for the existence of brain disease or the prediction of future outcomes (Arbabshirani, Plis, Sui, & Calhoun, 2017).
Functional Brain Connectivity During The Resting State
One way of measuring brain function is to use a non-invasive method, such as MRI or EEG, to observe the brain while a human individual performs an activity. This procedure is not always convenient in the context of biomarker discovery. One example is the ability of humans to learn and improve task performance which could interfere with diagnosis. There is also the difficulty for certain patients to perform tasks. For these reasons, observing the brain at rest, without the intervention of a particular task, is a promising approach for individual subject prediction which does not require individuals to perform a task (Greicius, 2008).
During a resting state data experiment, a person is requested to rest without performing any particular course of action. No particular consensus is yet adopted to determine whether eyes should be left open or closed. However, open eyes might help to reduce the possibility of sleeping. In many cases, a sight fixation item is used to aid the scanned person and in some cases eyes are monitored by staff outside the scanner. Through the analysis of collected data, a resting state brain connectivity pattern emerges. At this point, we can use analysis approaches to identify patterns in the brain activity that show activity consistent with the healthy brain (V. D. Calhoun & Adali, 2012; Fornito, Zalesky, & Breakspear, 2013). Abnormal deviations from a healthy connectivity pattern can indicate the presence of brain disease.
Two brain regions or networks can be connected in various ways. Anatomically, two regions can be linked by the physical extension of neurons called axons. Two brain regions can also show statistical similarity, that shows a functional connection. These connections are not necessarily active all the time. For example, closing our eyes when trying to remember could be a way of redirecting brain areas in charge of visual processing to aid by using visual memory images (Vredeveldt, Hitch, & Baddeley, 2011). In this context, if two brain areas are active at the same time then there is a type of connectivity due to a common function. If the activation is synchronized in time (activate and deactivate at the same time) then it is said that a functional connectivity is occurring (Buckner & Vincent, 2007; Van Dijk et al., 2010). Resting-state functional brain connectivity shows very similar patterns in individuals, although small variations in these patterns are known to be predictive of an individual person (Finn et al., 2015) or of brain disease (Arbabshirani et al., 2017).
Brain Functional Changes: Beyond Brain Structure
It might sound obvious that a strong direct hit to the head can damage the brain in such a way that certain capabilities are lost. However, in many cases, brain damage is not visible, even with advanced tomographic technology. Even in these mild cases, detriments in cognition and behavior can be observed (DeKosky, Ikonomovic, & Gandy, 2010). The fact that anatomical axonal connections might appear intact does not fully translate into a normal functionally connected brain. Resting-state functional connectivity changes after a brain injury incident in the mild cases have been shown to differentiate individuals with mild traumatic brain injury from healthy individuals.
The most notable change was an increase of functional connectivity between the cerebellum and brain areas in charge of processing movement (motor) (Nathan et al., 2015; Vergara, Mayer, Damaraju, Kiehl, & Calhoun, 2017). This increase of functional connectivity may be indicative of the brain to seek to compensate for the injury. Other diseases may also show both anatomical and functional brain changes as in schizophrenia (Skudlarski et al., 2010). Heavy alcohol consumption is both related to brain shrinkage (Kubota, 2001) and prominent brain disconnectivity (Vergara, Liu, Claus, Hutchison, & Calhoun, 2017; Vergara, Weiland, Hutchison, & Calhoun, 2017). These observations show that both types of connectivity can be complementary at providing diagnosis information.
Mining for Connectivity Features
Brain complexity makes it difficult to identify which functional connections are useful in identifying brain disease. The assumption that a single dysfunctional connection will characterize a brain disease is improbable given the collaboration of many parts of the brain to achieve functionality. On the other hand, an all-inclusive strategy may be degraded by noisy and confounding information. Data mining and machine learning techniques are powerful tools for separating useful from not-useful features.
This is important not only for diagnosis, but to understand the mechanism of a particular disease. Although results from machine learning can often overlap with regular statistical techniques, there is a chance that features rejected using confidence intervals or significance levels are in fact useful in identifying a brain disease. This is because it is often the case that combinations of abnormal connectivity are a better characterization of diseases than looking at a single connection (Chai, Walther, Beck, & Fei-Fei, 2009).
Many machine learning techniques are natively built to look at the influence of combined features in a multi-dimensional manner (Formisano, De Martino, & Valente, 2008). Given that different data modalities (e.g. MRI, genetic, chemical) can be extracted from the same subject with relative ease, several techniques have been developed to allow for a more precise characterization of brain diseases by fusing features from several modalities in one single analysis (V. D. Calhoun & Adali, 2009; Vince D Calhoun & Sui, 2016).
Summary
Technology is allowing for a better understanding of the brain in healthy and diseased states. The development and application of machine learning techniques to the huge amount of brain data that can be collected are crucial. The hope for the future is that machine learning will allow for a thorough analysis of the highly complex brain wiring. Detection of abnormal connections might be a key factor in the determination of early and actual diagnosis, as well as providing a better understanding of health risk factors. These abilities will allow for a better treatment and clinical outcomes of substance use, neurological disorders, and neuropsychiatric conditions.
References:
- Alzheimer’s, A. (2012). 2012 Alzheimer’s disease facts and figures. Alzheimers Dement, 8(2), 131-168. doi:10.1016/j.jalz.2012.02.001
- Arbabshirani, M. R., Plis, S., Sui, J., & Calhoun, V. D. (2017). Single subject prediction of brain disorders in neuroimaging: Promises and pitfalls. Neuroimage, 145(Pt B), 137-165. doi:10.1016/j.neuroimage.2016.02.079
- Buckner, R. L., & Vincent, J. L. (2007). Unrest at rest: default activity and spontaneous network correlations. Neuroimage, 37(4), 1091-1096; discussion 1097-1099. doi:10.1016/j.neuroimage.2007.01.010
- Calhoun, V. D., & Adali, T. (2009). Feature-based fusion of medical imaging data. IEEE Trans Inf Technol Biomed, 13(5), 711-720. doi:10.1109/TITB.2008.923773
- Calhoun, V. D., & Adali, T. (2012). Multisubject independent component analysis of fMRI: a decade of intrinsic networks, default mode, and neurodiagnostic discovery. IEEE Rev Biomed Eng, 5, 60-73. doi:10.1109/RBME.2012.2211076
- Calhoun, V. D., & Sui, J. (2016). Multimodal fusion of brain imaging data: A key to finding the missing link (s) in complex mental illness. Biological Psychiatry: Cognitive Neuroscience and Neuroimaging.
- Caroni, P. (2017). Neuro-regeneration: plasticity for repair and adaptation. Essays in Biochemistry, Volume 33: Molecular Biology of the Brain, 33, 53.
- Chai, B., Walther, D., Beck, D., & Fei-Fei, L. (2009). Exploring functional connectivities of the human brain using multivariate information analysis. Paper presented at the Advances in neural information processing systems.
- DeKosky, S. T., Ikonomovic, M. D., & Gandy, S. (2010). Traumatic brain injury—football, warfare, and long-term effects. New England Journal of Medicine, 363(14), 1293-1296.
- Finn, E. S., Shen, X., Scheinost, D., Rosenberg, M. D., Huang, J., Chun, M. M., . . . Constable, R. T. (2015). Functional connectome fingerprinting: identifying individuals using patterns of brain connectivity. Nat Neurosci, 18(11), 1664-1671. doi:10.1038/nn.4135
- Formisano, E., De Martino, F., & Valente, G. (2008). Multivariate analysis of fMRI time series: classification and regression of brain responses using machine learning. Magn Reson Imaging, 26(7), 921-934. doi:10.1016/j.mri.2008.01.052
- Fornito, A., Zalesky, A., & Breakspear, M. (2013). Graph analysis of the human connectome: promise, progress, and pitfalls. Neuroimage, 80, 426-444. doi:10.1016/j.neuroimage.2013.04.087
- Greicius, M. (2008). Resting-state functional connectivity in neuropsychiatric disorders. Curr Opin Neurol, 21(4), 424-430. doi:10.1097/WCO.0b013e328306f2c5
- Jablensky, A. (2000). Epidemiology of schizophrenia: the global burden of disease and disability. European Archives of Psychiatry and Clinical Neuroscience, 250(6), 274-285. doi:10.1007/s004060070002
- Kontis, V., Bennett, J. E., Mathers, C. D., Li, G., Foreman, K., & Ezzati, M. (2017). Future life expectancy in 35 industrialized countries: projections with a Bayesian model ensemble. The Lancet, 389(10076), 1323-1335. doi:10.1016/s0140-6736(16)32381-9
- Kowal, S. L., Dall, T. M., Chakrabarti, R., Storm, M. V., & Jain, A. (2013). The current and projected economic burden of Parkinson’s disease in the United States. Mov Disord, 28(3), 311-318. doi:10.1002/mds.25292
- Kubota, M. (2001). Alcohol consumption and frontal lobe shrinkage: study of 1432 non-alcoholic subjects. Journal of Neurology, Neurosurgery & Psychiatry, 71(1), 104-106. doi:10.1136/jnnp.71.1.104
- Murray, C. J. L., & Lopez, A. D. (1997). Alternative projections of mortality and disability by cause 1990–2020: Global Burden of Disease Study. The Lancet, 349(9064), 1498-1504. doi:10.1016/s0140-6736(96)07492-2
- Nathan, D. E., Oakes, T. R., Yeh, P. H., French, L. M., Harper, J. F., Liu, W., . . . Riedy, G. (2015). Exploring variations in functional connectivity of the resting state default mode network in mild traumatic brain injury. Brain Connect, 5(2), 102-114. doi:10.1089/brain.2014.0273
- Ross, C. A., Aylward, E. H., Wild, E. J., Langbehn, D. R., Long, J. D., Warner, J. H., . . . Tabrizi, S. J. (2014). Huntington disease: natural history, biomarkers and prospects for therapeutics. Nat Rev Neurol, 10(4), 204-216. doi:10.1038/nrneurol.2014.24
- Silberner, J. (2017, Monday, November 13, 2017). A Global State of Mind. Discover Magazine.
- Skudlarski, P., Jagannathan, K., Anderson, K., Stevens, M. C., Calhoun, V. D., Skudlarska, B. A., & Pearlson, G. (2010). Brain connectivity is not only lower but different in schizophrenia: a combined anatomical and functional approach. Biol Psychiatry, 68(1), 61-69. doi:10.1016/j.biopsych.2010.03.035
- Van Dijk, K. R., Hedden, T., Venkataraman, A., Evans, K. C., Lazar, S. W., & Buckner, R. L. (2010). Intrinsic functional connectivity as a tool for human connectomics: theory, properties, and optimization. Journal of neurophysiology, 103(1), 297-321.
- Vergara, V. M., Liu, J., Claus, E. D., Hutchison, K., & Calhoun, V. (2017). Alterations of resting state functional network connectivity in the brain of nicotine and alcohol users. Neuroimage, 151, 45-54. doi:10.1016/j.neuroimage.2016.11.012
- Vergara, V. M., Mayer, A. R., Damaraju, E., Kiehl, K. A., & Calhoun, V. (2017). Detection of Mild Traumatic Brain Injury by Machine Learning Classification Using Resting State Functional Network Connectivity and Fractional Anisotropy. J Neurotrauma, 34(5), 1045-1053. doi:10.1089/neu.2016.4526
- Vergara, V. M., Weiland, B. J., Hutchison, K. E., & Calhoun, V. D. (2017). The Impact of Combinations of Alcohol, Nicotine, and Cannabis on Dynamic Brain Connectivity. Neuropsychopharmacology. doi:10.1038/npp.2017.280
- Vining, E. P. G., Freeman, J. M., Pillas, D. J., Uematsu, S., Carson, B. S., Brandt, J., . . . Zuckerberg, A. (1997). Why Would You Remove Half a Brain? The Outcome of 58 Children After Hemispherectomy—The Johns Hopkins Experience: 1968 to 1996. Pediatrics, 100(2), 163-171. doi:10.1542/peds.100.2.163
- Volkow, N. D., Koob, G. F., & McLellan, A. T. (2016). Neurobiologic Advances from the Brain Disease Model of Addiction. N Engl J Med, 374(4), 363-371. doi:10.1056/NEJMra1511480
- Vredeveldt, A., Hitch, G. J., & Baddeley, A. D. (2011). Eye closure helps memory by reducing cognitive load and enhancing visualisation. Mem Cognit, 39(7), 1253-1263. doi:10.3758/s13421-011-0098-8
- Walker, E. R., & Druss, B. G. (2017). Cumulative burden of comorbid mental disorders, substance use disorders, chronic medical conditions, and poverty on health among adults in the U.S.A. Psychol Health Med, 22(6), 727-735. doi:10.1080/13548506.2016.1227855
- Zaloshnja, E., Miller, T., Langlois, J. A., & Selassie, A. W. (2008). Prevalence of long-term disability from traumatic brain injury in the civilian population of the United States, 2005. J Head Trauma Rehabil, 23(6), 394-400. doi:10.1097/01.HTR.0000341435.52004.ac
These findings are described in the article entitled The effect of preprocessing in dynamic functional network connectivity used to classify mild traumatic brain injury, published in the journal Brain and Behavior. This work was led by Victor M. Vergara & Vince D. Calhoun from The Mind Research Network and the University of New Mexico.