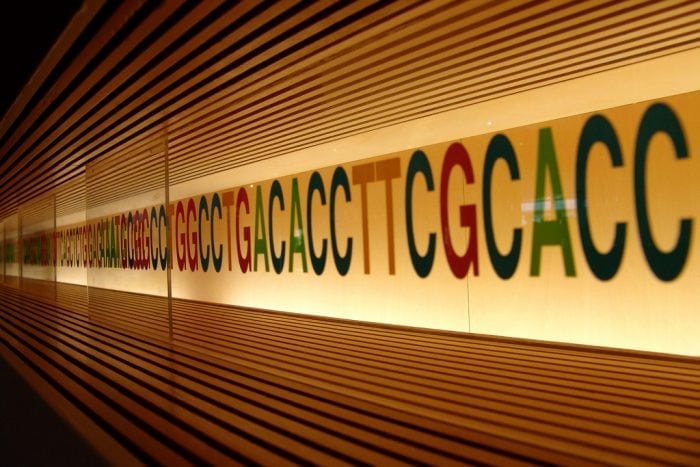
How strongly do our genes contribute to type 2 diabetes risk? Are heart disorders more heritable among men than women? And is there a shared genetic basis for schizophrenia and depression? The explosive growth of genetic data provides unprecedented opportunities to answer such questions.
At the heart of modern genetics lies the observation that most common diseases are polygenic, meaning there is no single mutation acting as a risk factor. Rather, disease risk arises due to the aggregate effect of thousands of genetic variations, each one exerting a tiny effect on its own. Hence, diseased individuals likely carry thousands of genetic variations contributing to disease susceptibility.
Although polygenic diseases are extremely complex biologically, some of their key properties are surprisingly easy to understand. This is a result of the huge number of genetic variations, which enables finding statistical patterns distinguishing cases (i.e., diseased individuals) from controls (healthy individuals) via “black-box” techniques, without fully understanding the underlying biology. More concretely, statistical geneticists use probabilistic models that accumulate numerous small signals to identify the overall signal; this accumulation turns out to be easier than identifying and measuring each small signal separately. As an analogy, it is often easier to predict the mass action of many gas molecules than the trajectory of a single molecule, due to large-sample dynamics.
In recent years, researchers have employed such black-box techniques to understand two key properties of genetic diseases: Genetic heritability — the degree to which disease risk is affected by genetic versus environmental factors — and genetic correlation, which measures the genetic similarity between diseases. The latter quantity is arguably more interesting because it can expose surprising biological relationships between diseases, such as schizophrenia and anorexia nervosa. These biological relationships can in turn help to better understand genetic diseases and guide drug development.
While genetic studies have tremendous scientific value, they also involve genetic privacy risks. Our genomes can reveal much about ourselves, including our ancestry, our relatives, and our disease risks. Such information is valuable for genetic research, but it can also be used for potentially sinister purposes such as job discrimination. Consequently, legal and logistical concerns prevent the sharing of genetic data between genetic researchers. This imposes a severe limitation on genetic correlation studies because different genetic diseases are often studied in different scientific institutes, but the data cannot be shared.
Fortunately, there exist elegant ways to investigate genetic correlation without genetic data sharing. The underlying idea is that several scientific institutions can each collect genotypes and disease data from a sample of individuals, and then share summary statistics that do not expose the raw genetic data. These summary statistics allow estimating genetic heritability and correlation in a privacy-preserving manner. While this technique sounds like magic, it is based on simple mathematical principles.
To understand the underlying idea, we first need to understand standard black-box methods. Recall that DNA is a sequence of the letters “A,” “T,” “C,” and “G.” We assume that every position in the sequence wherein different individuals have different letters (i.e., a genetic variant) exerts a small effect on the studied trait. Taking height, for example, a certain letter can increase height by 0.001cm on average if it is “A,” or decrease it by 0.001cm on average if it is “G.” Under this assumption, we can estimate genetic correlation between two traits (e.g. height and blood pressure) roughly as follows: First, we convert the measurements of the two traits to have the same average and scale. Next, we compute two quantities for every pair of individuals — the difference between their transformed measurements and the number of differences between their DNA sequences. Finally, we examine if the first quantity tends to be small when the second quantity is small. Such a co-occurrence indicates a strong genetic correlation.
How can the above technique be employed in a privacy-preserving manner? Let us start with a simplified example. Imagine that two researchers each observes a sequence of numbers that the other can’t see. The researchers wish to calculate the total average of the two sequences, without revealing the actual sequences. Each researcher reports the sum and length of the sequence to the other one. The total average is the sum of the reported sums, divided by the sum of the reported lengths. The key idea behind privacy-preserving black-box methods is similar. The genetic correlation formula can be written in the form a1b1 + a2b2 + … + anbn, where the a quantities depend only on data from study 1, and the b quantities depend only on data from study 2. Hence, two research institutes can each report the corresponding a or b values without exposing raw genetic data.
The above technique is perfectly suitable for continuous traits such as height and blood pressure, but it cannot be directly applied to genetic diseases, where the trait values can take two possible values: Case or control. Several previous studies used the above technique with the encoding control=0, case=1. However, this rough approximation can be very inaccurate in practice.
Another critical aspect of most disease studies is that the analysis is not applied to a random sample of individuals from the population, because even so-called common diseases are relatively rare. For example, for a disease affecting 1% of the population (e.g. schizophrenia), a random sample of 1000 individuals would include only 10 cases. Rather, these studies use case-control sampling, where cases of the disease are significantly over-sampled compared to their population prevalence, typically yielding about 50% cases in the study. As we show in our paper, it is critical to take this sampling approach in designing methods to estimate genetic correlation, or the results may be very misleading.
To close these gaps, we formulated a new type of summary statistics that are designed for genetic diseases. Our formulation exploits the fact that the formula for genetic correlation between genetic diseases depends on the kinship between pairs of individuals. Briefly, kinship is a measure of genetic relatedness and is equal to 50% for siblings, 25% for cousins, and so on. The kinship values in typical studies are close to zero because such studies exclude related individuals. Our formulation exploits this observation by using a celebrated mathematical technique called the Taylor expansion, which can approximate a complex mathematical function using a simpler one. In its simplest form, the Taylor expansion of a function of the form y = f(x) yields the approximate function y = ax + b, where a and b are the two numbers yielding the best approximation for small values of x. Although the approximation is generally poor, it can be remarkably accurate when x is close to zero. By applying the Taylor expansion to genetic correlation, we were able to obtain an accurate approximation that depends solely on privacy-preserving summary statistics.
The main analysis in our paper examined genetic correlation between schizophrenia and bipolar disorder. We estimated the correlation to be ~43% — a number that is over 20% smaller than reported in previous studies. We additionally found a putative correlation between rheumatoid arthritis and coronary artery disease that has not been demonstrated before.
Overall, we developed a new method that allows research institutes to collaborate to estimate genetic correlations between diseases without compromising genetic privacy. Such collaborations can improve our understanding of genetic diseases and help find unexpected relationships between genetic traits. Our results suggest that schizophrenia and bipolar disorder may not be as genetically similar as previously thought and that a careful analysis can often lead to vastly different results than those obtained under previous approaches, that do not fully account for the case-control nature of the study.
These findings are described in the article entitled Estimating SNP-Based Heritability and Genetic Correlation in Case-Control Studies Directly and with Summary Statistics, recently published in the American Journal of Human Genetics. This work was conducted by Omer Weissbrod and Saharon Rosset from Tel Aviv University, and Jonathan Flint from the University of California Los Angeles.