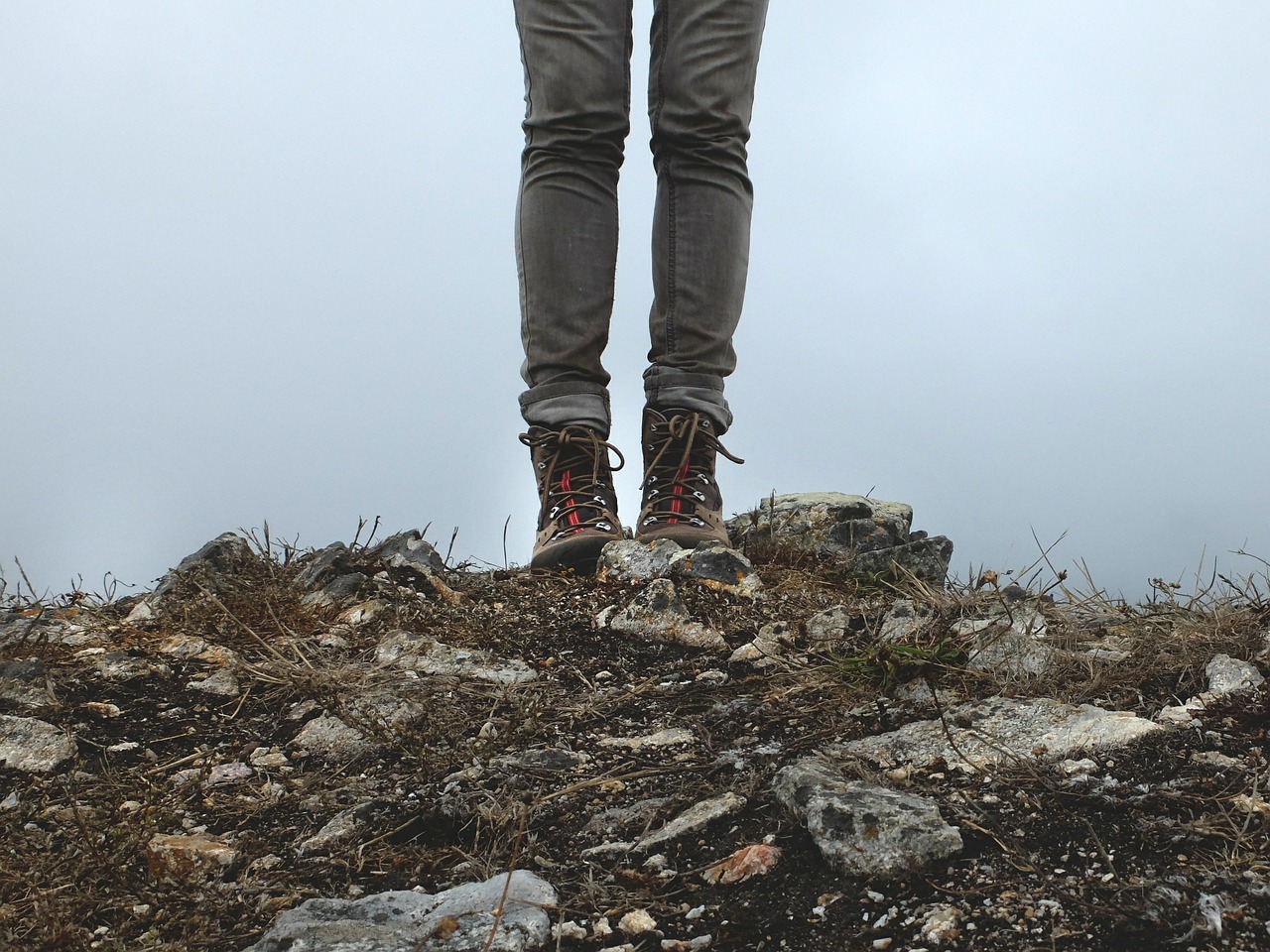
The scientific community relies on the projections of Earth system models (ESMs) to evaluate the response of the earth to climate change, including a positive feedback loop between soil carbon (C) and climate, where accelerated decomposition of soil organic matter (SOM) under warming further exacerbate climate change.
However, soil heterotrophic respiration, Rh (or, microbial processing of SOM to CO2), one of the biggest ecosystem fluxes of carbon dioxide (CO2), in these models is based on very simplistic temperature response function, known as Q10. Sometimes this Q10 temperature function is accompanied by a soil moisture scalar. These soil moisture scalars are often derived by empirically fitting site-specific soil moisture data with Rh using non-linear (polynomial and parabolic) regression equations. This partly explains why ESMs often fail to capture the full range of spatial and temporal variations in soil fluxes, which reduces the predictability of these models.
The Dual Arrhenius and Michaelis-Menten (DAMM) model simulates Rh using Michaelis-Menten (an enzyme kinetic process where rates of reaction follows a hyperbolic relation with the substrate), Arrhenius (an exponential temperature function), and diffusion (transport of solute and gas through water-filled and air-filled pore space in soil) functions (Davidson et al., 2012, Fig. 1).
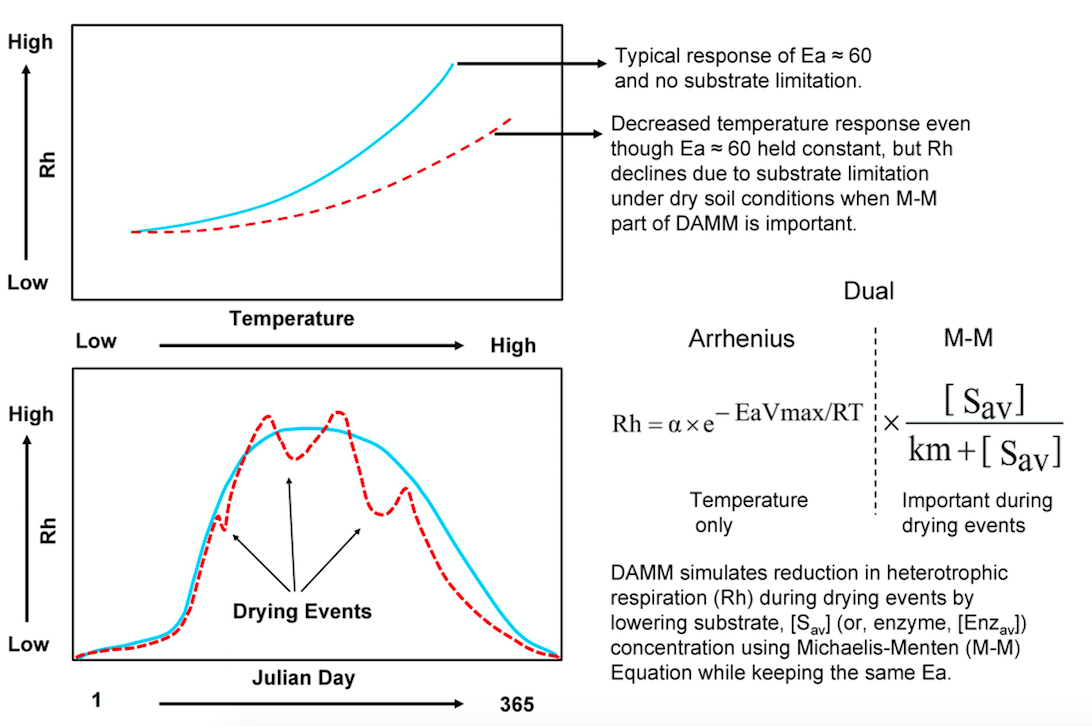
Fig. 1: Explicit representation of soil moisture effects on soil heterotrophic respiration in Dual Arrhenius and Michaelis-Menten (DAMM) model. Reproduced from Sihi et al. (2018) with copyright permission from ELSEVIER.
Soil moisture affects Rh and its apparent temperature sensitivity (the observed temperature response of Rh) in DAMM by regulating the diffusion of soluble-C substrates at low moisture and diffusion of oxygen at high moisture contents. A recent study showed how the DAMM model can be easily merged with a larger ecosystem model (Forest Biomass, Assimilation, Allocation and Respiration, FöBAAR; Keenan et al., 2012), replacing its overly simplistic Q10 functions, and thereby improve the performance of the ecosystem model (Fig. 2). By doing so, they intend to demonstrate how other ecosystem models and ESMs could also adopt their approach.
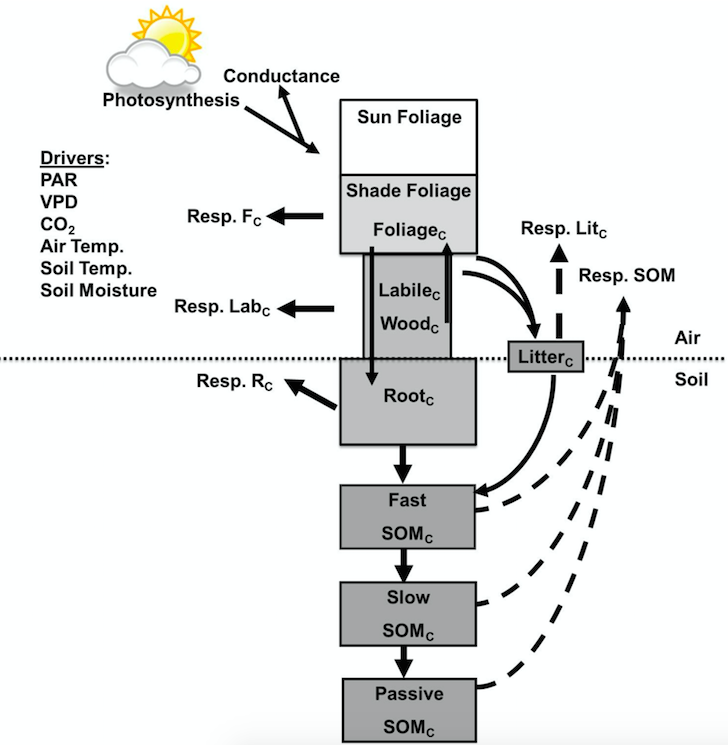
Fig. 2: Integrating DAMM (Dual Arrhenius and Michaelis-Menten, Davidson et al., 2012) soil flux model into FöBAAR (Forest Biomass, Assimilation, Allocation and Respiration, Keenan et al., 2012) ecosystem flux model. Dashed arrows represent CO2 fluxes from litter and three soil organic matter Pools (fast, passive, and slow pools), where we replaced FöBAAR’s algorithms for soil heterotrophic respiration with those of DAMM. Reproduced from Sihi et al. (2018) with copyright permission from ELSEVIER.
Sihi et al. (2018) used identical datasets (high-frequency soil flux data from automated soil chambers, landscape-scale ecosystem fluxes from eddy covariance towers, and other campaign-based measurements like litterfall and leaf area index) at two AmeriFlux sites in the northeastern USA (Harvard Forest, MA and Howland Forest, ME) to calibrate the merged (DAMM-FöBAAR) and the unmerged (FöBAAR-only) models, using an iterative process (Williams et al., 2009). Soil flux from root-exclusion (trenching) experiment was used to directly parameterize the Rh component of the DAMM-FöBAAR model. The performance of both DAMM-FöBAAR and FöBAAR-only models were then validated for short-term (seasonal) and long-term (decadal) soil (sum of soil microbial and root respiration) and ecosystem (sum of autotrophic and heterotrophic respiration) fluxes. The calibrated DAMM-FöBAAR model reduced the model-data mismatch for seasonal and decadal-scale soil (and ecosystem) respiration compared to the FöBAAR-only model for both sites, with a significantly improved performance under conditions with extreme soil moisture variations (e.g. drying events).
Above findings indicate that more explicit representation of soil moisture (and substrate supply) effect of Rh in the DAMM-FöBAAR model is robust across time and space. More importantly, the Michaelis-Menten equations in the DAMM model control the substrate-C limitation under drying (and oxygen under flooding) conditions, while keeping the temperature sensitivity parameter constant (i.e. the “Ea” in the Arrhenius function, Fig. 1). This is a big advantage over the models with classical Q10 representations such that the Q10-based models would not be able to reproduce soil respiration under drying (or flooding) events unless it is calibrated under similar environmental conditions. Hence, Sihi et al. (2018) have demonstrated that the DAMM model equations increase the predictive capacity of soil (and ecosystem) respiration with a relatively minor additional model complexity.
Finally, the simple yet more explicit representation of soil moisture effects on substrate supply for Rh in the DAMM model equations should be more generalizable than the wide variety of empirical regression equations commonly used and could be easily incorporated into bigger ESMs.
These findings are described in the article entitled, Merging a mechanistic enzymatic model of soil heterotrophic respiration into an ecosystem model in two AmeriFlux sites of northeastern USA, recently published in the journal Agricultural and Forest Meteorology.
This work was conducted by Debjani Sihi (currently at ORNL) and Eric A. Davidson from the University of Maryland Center for Environmental Science, Min Chen from the Pacific Northwest National Laboratory, Kathleen E. Savage from the Woods Hole Research Center, Andrew G. Richardson from Harvard University and Northern Arizona University, Trevor F. Keenan from Lawrence Berkeley National Laboratory, and David Y. Hollinger from the USDA Forest Service.
References:
- Davidson, E.A., Samanta, S., Caramori, S.S., Savage, K., 2012. The dual arrhenius and michaelis–menten kinetics model for decomposition of soil organic matter at hourly to seasonal time scales. Global Change Biollogy 18, 371–384.
- Keenan, T.F., Davidson, E.A., Moffat, A.M., Munger, W., Richardson, A.D., 2012. Using model-data fusion to interpret past trends, and quantify uncertainties in future projections, of terrestrial ecosystem carbon cycling. Global Change Biology. 18, 2555–2569.
- Sihi, D., Davidson, E.A., Chen, M., Savage, K.E., Richardson, A.D., Keenan, T.F., Hollinger, D.Y., 2018. Merging a mechanistic enzymatic model of soil heterotrophic respiration into an ecosystem model in two AmeriFlux sites of northeastern USA. Agricultural and Forest Meteorology 252, 155–166.
- Williams, M., et al., 2009. Improving land surface models with FLUXNET data. Biogeosciences 6, 1341–1359.