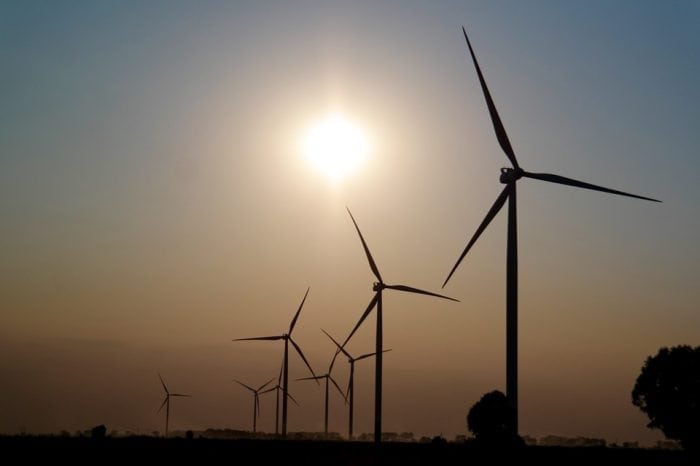
The past decade has seen a rapid increase in the growth of wind energy in the United States. While conversion to renewable energy sources is necessary to reduce worldwide CO2 emission, the intermittency of wind energy creates a new set of challenges to be addressed. Specifically, the power output of a wind farm is not steady but fluctuates as the wind speed fluctuates, with fluctuations spanning time periods ranging from months to minutes.
The electric grid was designed to only handle small amounts of varying power production, so this problem will only become more difficult to handle as more and larger wind farms are built. This intermittency is currently mitigated by filling in the periods of decreased wind with increased energy production primarily from natural gas power plants, which, while cleaner than coal and oil, are still a significant source of CO2 emissions.
A team of researchers at The University of Vermont has been working to address this intermittency problem as part of a larger National Science Foundation funded program aimed at addressing the public policy, behavioral, and technological aspects of the next-generation electric grid. They believe that through the use of more advanced control systems, wind farms will be able to supply a steadier and more controllable power input to the electric grid. In a study recently published in the Journal of Wind Energy, the team examined the effect of multiple time scales on a wind farm controller working to both maximize the energy produced, while simultaneously minimizing the power fluctuations from a wind farm.
Using Model Predictive Control To Maximize Wind Turbines
Traditional wind farm control techniques focus on maximizing individual wind turbine energy output through proportional-integral-derivative (PID) controllers. These systems cannot take into account the energy produced by neighboring wind turbines nor account for how the wind speed might change in the future. Instead of these traditional control techniques, the team chose to employ a technique called model predictive control (MPC) for their study. MPC techniques have the ability to handle multiple objectives and incorporate a time horizon into their solution allowing a single controller to take into account both the instantaneous power produced by multiple wind turbines to account for the change in the wind farm power production based on a prediction of how the future wind speed will change.
The team first discovered that the power fluctuations from a wind farm depend greatly on the ratio of the time it takes for a wind gust to travel from one turbine to a downstream turbine to the dominant frequency of the fluctuating wind gusts. For certain values of this ratio, the power outputs from individual turbines produce constructive interference, leading to farm-level power fluctuations that are greater than any single turbine. For other values, the individual turbine power outputs produce destructive interference, causing the farm level power fluctuations to be significantly smaller than the fluctuations from the individual turbines.
The team then discovered that a wind farm controller, working to both simultaneously maximize the electric energy output from the wind farm and minimize the power fluctuations, could significantly reduce the power fluctuations with only minimal reductions to the total farm power output when given a prediction of how the wind speed will change in the future. The method utilized to achieve these power reductions was sensitive to the form of the objective function within the controller. If the objective function was formulated to minimize the time derivative of the power fluctuations, the controller smoothed the power curves of the individual wind turbines. If the objective function was formulated to minimize the variance in farm power relative to the average power output, the controller smoothed the total farm power by utilizing constructive and destructive interference effects between the individual wind turbines.
The results of this study indicate that model predictive control techniques have the potential to make wind farms a more reliable, controllable resource for electricity generation. Understanding the effect of the different time scales involved in a wind farm is critical to designing an effective controller. Improving methods of wind speed forecasting, as well as the mathematical models used for wind farm controllers, will play a significant role in making this technology reliable and practical as an ever greater amount of energy is produced from wind farms.
This study, The effect of timescales on wind farm power variability with nonlinear model predictive control was recently published in the journal Wind Energy.