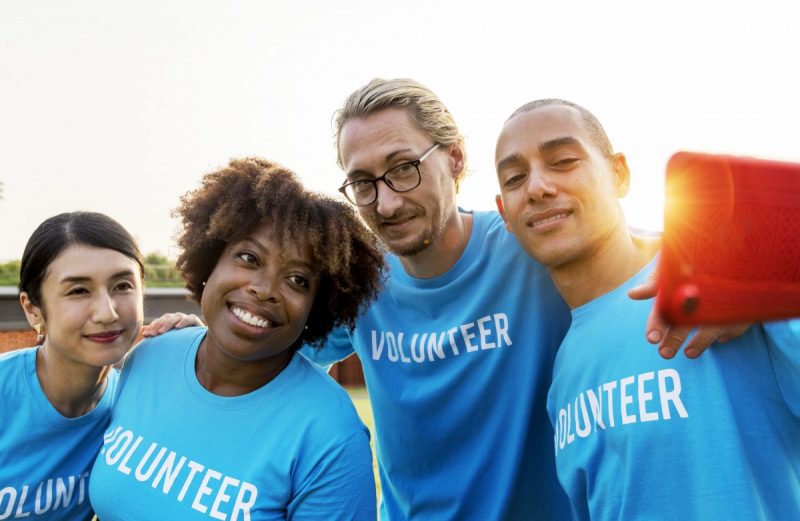
Across the human genome, there are a few million base pairs that differentiate one person from another. Though these differences account for only 0.1% of the total genome, they affect many of our traits, such as eye color, propensity for diseases, or even tea drinking habits. In the numerous genetic studies in humans over the past few decades, however, the vast majority of genetic studies have included only people of European descent, creating a disparity in the benefits of research for non-Europeans.
These few million DNA differences are known as single nucleotide polymorphisms, or SNPs. Of the SNPs that have been shown to associate with diseases, many are located in between genes rather than in the regions of genes that code for proteins. Many of these “in-between” SNPs associate with gene expression (RNA) levels and thus one mechanism for how SNPs lead to differences in disease susceptibility is through differences in gene expression regulation. The differences in our genome, though small, have aggregate effects on larger traits like disease susceptibility or drug response. However, like most genetic studies, DNA-RNA association studies have mostly been performed in solely European populations.
In our paper “Genetic architecture of gene expression traits across diverse populations,” recently published in PLOS Genetics, we investigated how genetic diversity affects gene expression. We used data from the Multi-Ethnic Study of Atherosclerosis (MESA) to study the underlying genetic architecture of gene expression by optimizing gene expression prediction within and across diverse populations. MESA includes self-identified individuals of African American (AFA), Hispanic (HIS), and European (CAU) ancestry.
Populations such as African American and Hispanic also pose unique insights into genetic admixture, which occurs when two or more previously isolated populations mate, due to the history of colonization and slavery in the Americas. We built statistical models that tested genotypes (DNA) for association with gene expression (RNA) and make our results publicly available, allowing future studies of diverse populations to predict gene expression from genotype.
We compared our findings to those in other cohorts and found that among similarly sized cohorts, the replication rate was best in cohorts with the most similar ancestry, such as AFA with a Nigerian cohort and HIS with a Mexican cohort. However, the Nigerian and Mexican cohorts were much smaller than the European replication populations we also tested. Replication rates were higher in the larger sample sized European populations due to more statistical power, indicating the need for more diverse samples. Between MESA populations, genetic correlation was also highest between the two populations with the most similar ancestry, HIS and CAU, with correlation increasing as the heritability of gene expression increases.
We found that differences in predictive performance between our models arise from allele frequency differences between populations. By comparing genes with predictive performance differences between populations, we found that gene models with a larger difference in predictive performance comprise SNPs with greater differences in allele frequencies between populations. We also identified a class of genes where predictive performance drops substantially between populations, which would not have been found with solely European predictors. Thus, it may be beneficial to build gene expression prediction models using training populations with a similar allele frequency spectrum to that of the planned test cohort taking into account SNPs that are interrogated in both populations. The inclusion of diverse populations in complex trait genetics is crucial for the equitable implementation of precision medicine.
These findings are described in the article entitled Genetic architecture of gene expression traits across diverse populations, recently published in the journal PLOS Genetics. This work was conducted by a team including Angela Andaleon and Heather Wheeler from Loyola University Chicago.