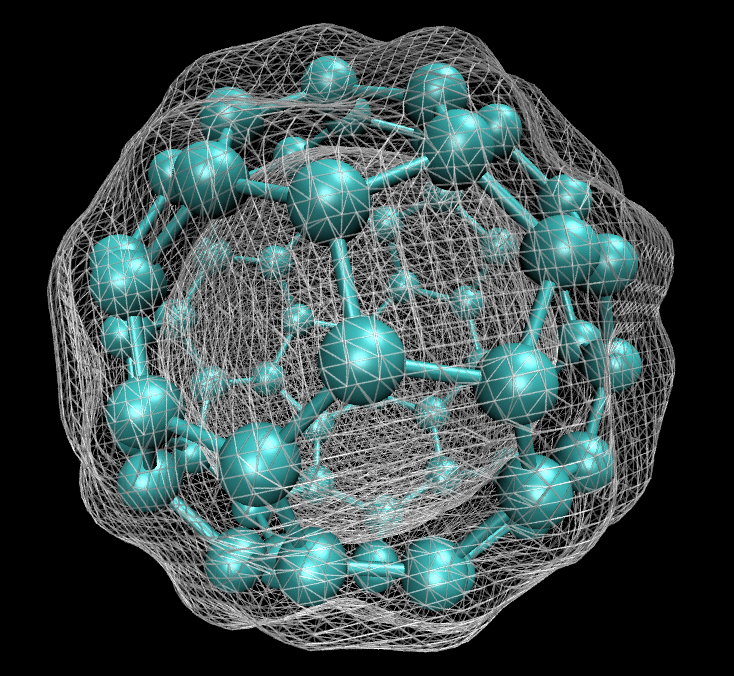
Electrons whizzing around an atom and a group of people at a concert hardly seem to have much in common. So, it comes as a surprise that researchers at Cornell have found a way to accurately model the behavior of groups of organisms using mathematical models originally developed for quantum mechanics.
In a study published August 30th, the group of researchers introduced a mathematical framework to model population behavior that is based on Density functional theory (DFT), a mathematical modeling technique used in physics and chemistry to describe the properties of many-bodied quantum systems. By using a modified version of DFT the team was able to correctly predict the large-scale behavior of fruit fly populations in new environments. The team is confident that their framework can be extended to model the behavior of human populations as well.
Such a finding is important as it both provides a computationally elegant and efficient way to model the behavior of large groups of organisms and it also elucidates an unexpected connection between the realms of quantum mechanics and population biology. According to Tomas Arias, one of the authors on the study, “This is one of the all-too-rare cases – particularly where living systems are involved – where the theory preceded the experiments, and the experiments, in precise mathematical detail, completely confirmed the theory.”
DFT, Systems, And Fruit Flies
Modeling the behavior of large groups of things can be hard. Common sense would seem to dictate that the most accurate way to model a group of things would be to model each thing individually and how it interacts with others in the group. In some cases this “bottom-up” approach makes sense. But, when you are working with extremely large groups of things, modeling the dynamics of each member of that group would result in a ridiculously complex and burdensome model. DFT is an important modeling technique as it allows one to describe the properties of a multi-body system without having to compute the properties and dynamics of each individual body in that system, saving a lot of time, computational power, and headaches.
In the context of quantum electrodynamics, the main parameter that informs DFT models is electron density—that is, a measure of the average electron distribution over an area of space. If one knows the electron density of a particular system, then one can infer other information about that system, such as its ground-state properties. The general conceit of DFT is that the global properties of a system can be determined even without knowing all the properties of the individual things in that system.
By examining the behavior of groups of fruit flies, the researchers extracted two functional operators; one meant to quantify how much the flies are attracted to different parts of their environment—the “vexation” function, and the other to quantify the degree of crowding the fruit flies will endure in a particular spot—the “frustration” function. These two functional operators are the fundamental parameters of the model, just like how electron density is the fundamental parameter of DFT models used in quantum mechanics. The relative preferability of a location is the sum of vexation and frustration effects over that location.
By using these derived functional equations, the researchers were able to predict the collective behavior of groups of fruit flies in new environments. The flies groups around locations that had a high relative preferability and tended to avoid areas with low preferability. Moreover, the scientists were able to quantify the overall “mood” of the population, which could be used to predict how the population distribution would change over time. Biologists for decades have been constructing “top-down” models of population behavior, but as of now, there is no generally accepted method for predicting the emergent collective behavior of living organisms. This new model could represent a step in that direction.
According to Arias, fruit flies were chosen as a test subject as they, and their close cousin the housefly, are a “convenient and ethical first test system.” However, the researchers think that this method could be extended to analyze the behavior of human collectives and be used to predict things like the crowd distribution at political rallies, patterns of immigration, or population distributions in cities.
Insights For Modelling In the Sciences
The applicability of DFT to population biology represents an interesting feature of scientific models; the same model can often be used to describe multiple target systems that have different physical natures. The underlying dynamics responsible for electron behavior are most certainly different than the dynamics underlying the collective behavior of organisms, but we can nonetheless use the same class of mathematical models to accurately describe both phenomena. This multi-faceted applicability of DFT successfully unites a diverse group of phenomena under a single class; a hallmark of scientific progress since Newton.
Additionally, such a model shows that it is entirely possible to determine the global properties of a system without saying much about the individual constituents of that system. The traditional understanding of scientific modeling may hold that the most accurate and useful models are the ones that veridically represent the target system with a 1-to-1 correspondence between elements of the system and elements of the model. In other words, traditional understanding believes that when it comes to scientific models, the more details, the better. On the contrary, we can sometimes gain a more accurate understanding of a system by abstracting away from specific details. In some cases, predictive power and understanding can result from deliberately misrepresenting the target system, as is the case when scientists introduce fictional idealizations like frictionless surfaces or continuous fluids.
Lastly, such a study illustrates the blossoming integration of mathematical and computational methods into the biological sciences. For quite a while, the biological sciences have been considered “behind” the other physical sciences, due to the former’s relative lack of rigorous mathematical formalisms. The introduction of techniques common to quantum mechanics into the realm of biology signifies a kind of dissolution of methodological barriers that have traditionally divided scientists. It hints at the bright future of science as an explicitly interdisciplinary process that can confront problems that span across multiple domains.