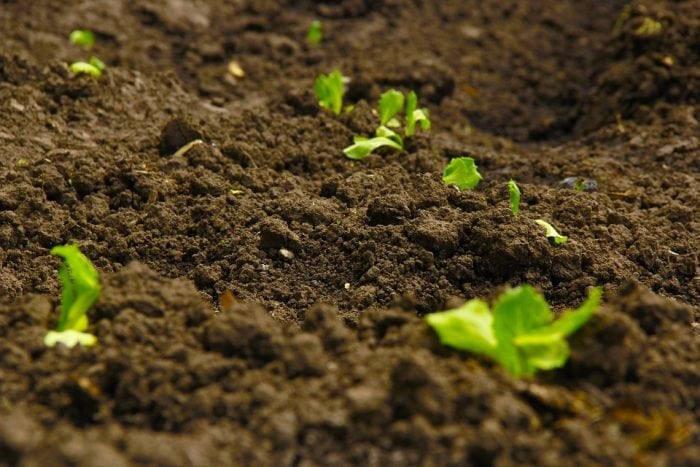
Soil sustains life by the virtue of the nutrients and moisture it holds. Predicting the dynamics of soil moisture is of great importance for agricultural or water resource management. However, predicting soil moisture is a challenging task given the requirement of large-scale, complex numerical models, which are based on dataset like initial conditions, soil texture, vegetation, atmospheric forcings (radiation, wind, temperature, precipitation, etc.), topography, etc.
Using this dataset and simulating accurate estimates of soil moisture for a sustained period requires reliable models and dataset. Expert skills in model development, application, and analysis are also equally crucial. Moreover, most common hydrological models are calibrated based on the retrospective dataset without accommodation of changes in climate, thus assuming a stationary rainfall-runoff relationship. This would lead to uncertainty (and hence, error) in the estimation of soil moisture dynamics due to implementation, design, and application of the models.
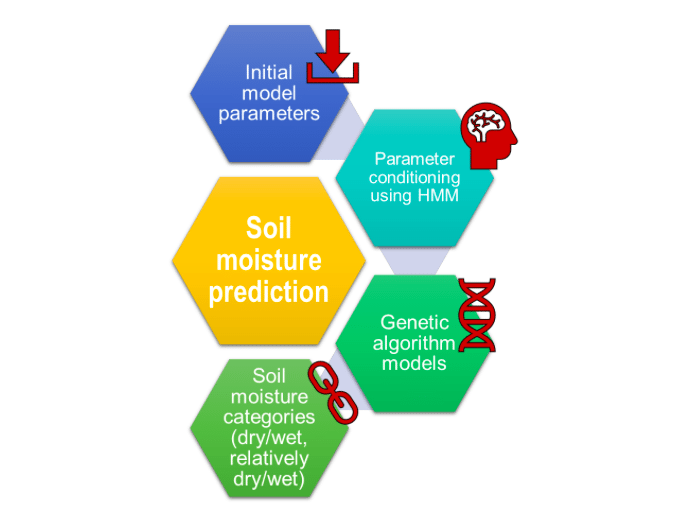
A simple representation of the proposed approach for forecasting soil moisture dynamics. Republished with permission from Elsevier from: https://doi.org/10.1016/j.jhydrol.2018.07.003
A new algorithm is proposed by integrating a stochastic Hidden Markov Model (HMM) with genetic algorithm (GA), an evolutionary algorithm based on the process of natural selection, in order to provide a robust framework to predict soil moisture dynamics reliably across different climatic regions. The algorithm is tested for two locations in the U.S — Iowa and Illinois — to test the transposability of the approach.
The HMM adds the “smart” component to the approach by providing better-trained parameters for obtaining the weather states through optimized initial parameters derived by a GA. The HMM conditions the input parameters required for a model to estimates the weather states of the soil (e.g., Wet, dry, relatively dry or relatively wet). These initial conditions help the GA-based approach to better forecast the soil moisture values using precipitation dataset and historical statistics derived from the antecedent soil moisture values. This coupling eliminates the need for using a parameter intensive physical model and minimizes not only the user’s skills in determining initial parameter values, but also model structural (i.e., parameterizations, initial/bottom boundary conditions, governing equation, transposability, etc.) and measurement (i.e., land covers, soil information, etc.) errors.
To test the results, the soil moisture predictions derived by this approach were compared with those from a sophisticated near-surface soil moisture assimilation scheme based on a physically based inversion model (IM) (Ines and Mohanty, 2008a) called Soil-Water-Atmosphere-Plant (SWAP) model. The results indicate consistent improvement in the predictive accuracy by using the proposed approach compared to SWAP-GA method. The time-averaged (1994–2000) soil moisture at the near-surface (0–10 cm) and in the sub-surface layers (10–30, 30–50, and 0–50 cm) were predicted at multiple locations and at the various soil depths in the study area, which matched well with the in-situ measurements.
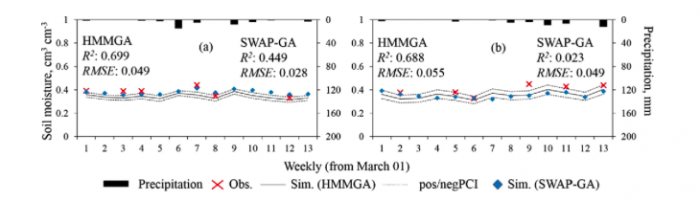
Comparison of the results from the proposed stochastic model (HMMGA) with a sophisticated physically-based model (SWAP-GA) to demonstrate the advantage of using the new algorithm over other complex models. Republished with permission from Elsevier from: https://doi.org/10.1016/j.jhydrol.2018.07.003
This is the first application of a stochastic model for forecasting long-term soil moisture dynamics using other proxies like precipitation at multiple spatio-temporal domains across the land surface. The results of this pilot study are very encouraging and open up the path of predicting soil moisture at large spatial scales, especially in conjunction with satellite-derived soil moisture (AMSR-E, AMSR2, SMOS, SMAP, etc.) and precipitation (TRMM/GPM) dataset.
Despite uncertainties at a daily time step, the new approach could provide relatively reliable model outputs at the weekly time intervals and relatively good transposability under different weather conditions. This “smart” approach provides easy convenience (avoiding intensive input parameters for physically-based hydrological models) in application to fields and minimizes not only user’s skills in determining initial parameter values, but also model structural (i.e., parameterizations, initial/bottom boundary conditions, governing equation, transposability, etc.) and measurement (i.e., land covers, soil information, etc.) errors.
These findings are important, as the proposed methodology can provide an attractive alternative in forecasting long-term soil moisture for the future using only climate change scenarios (i.e., global climate models-GCMs, regional climate models-RCMs, etc.) and historical soil moisture measurements (available). In this regard, this approach could be quite useful for developing/ improving efficient agricultural/water resources management in the future.
These findings are described in the article entitled Development of non-parametric evolutionary algorithm for predicting soil moisture dynamics, recently published in the Journal of Hydrology. This work was conducted by Yongchul Shin from Kyungpook National University, and Binayak P. Mohanty and Amor V.M. Ines from Texas A&M University.