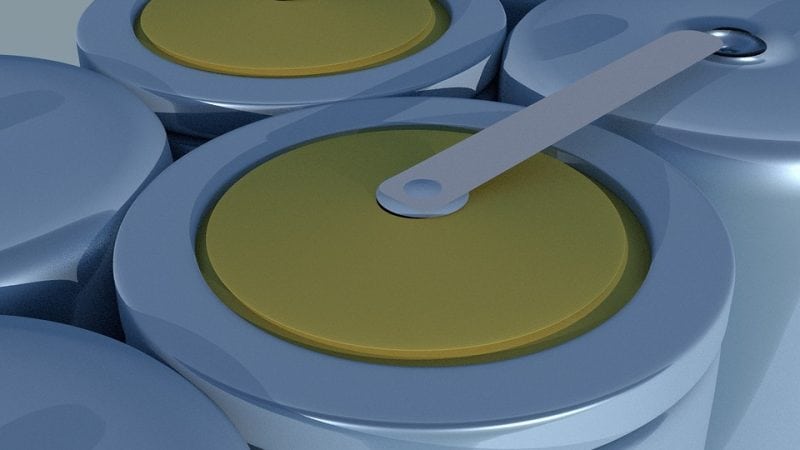
Environmental pollution and energy crises make people develop new energy. Lithium-ion batteries, which have the characteristics of long cycle life, low self-discharge rate, and environmental friendliness, are widely applied in energy storage systems [1]. To ensure the battery works with high efficiency, an accurate estimation of battery state of energy (SOE) and state of power (SOP) is necessary.
SOE is commonly defined as the ratio of the residual energy to the maximum available energy [2], which is the function of battery load current and terminal voltage [3]. The SOP is employed to reflect the battery power capability, which is a key parameter to ensure the battery work in a safe region. It cannot be measured by measuring equipment directly. The common method of peak power prediction was hybrid pulse power characterization method (HPPC).
In general, a battery is made up of a lot of single cells. The differences in cells’ parameters and working temperatures lead to the cells’ inconsistency and make the method of battery SOE and SOP difficulty. To solve these problems, the equivalent circuit battery model is first applied to reflect dynamic behavior of a battery. And then, the method of particle swarm optimization (PSO) is applied in battery model parameters identification while the battery SOE and terminal voltage are predicted by unscented Kalman filter (UKF). Besides, the SOP is determined by cells’ terminal voltage, SOC, and design limit. The proposed method is simple and can be implemented on a microcontroller.
In the method of PSO-UKF, accuracy history data is first stored in a microcontroller used for battery model parameters identification by PSO. Based on the accurate battery model parameters, the UKF is applied in battery SOE and SOP estimation at each micro time. Besides, the history data is updated during the process of UKF. Due to the battery model parameters changing slowly, it is only updated at each macro time.
Based on above analyzation, the proposed method only refers to simple arithmetic operation without complex matrix operation. And battery model parameters identification based on the method of PSO is not running all the time. Therefore, the proposed method can be applied for real-time battery pack SOE and SOP estimation conducted on a microcontroller. Besides, the characteristics of battery maximum available capacity and open-circuit voltage (OCV) are analyzed under different working temperatures (see Fig. 1).
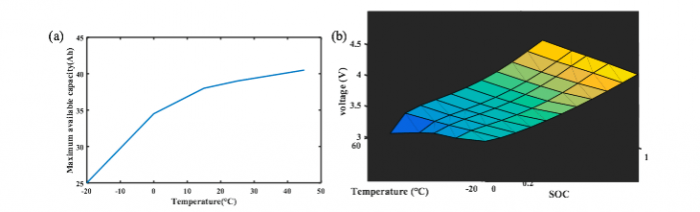
Fig. 1 Cell characteristic test results under different temperatures: (a) Maximum available capacity; (b) OCV-SOC test results. (Image courtesy of Chen Zonghai)
To verify the accuracy and robustness of the proposed method, the method of RLS-UKF in reference [4] is employed to compare with the proposed method in battery SOE and SOP estimation under the NEDC profiles and dynamic profiles. The experimental results show that the battery peak discharge/charge current is mainly determined by cell voltage and battery consistency and the battery available energy changes with the battery working temperature which leads to the battery available energy increase with the cells’ working temperature growth. Besides, the battery inconsistency in cells working temperatures lead to the SOC unconformity and restricts the upper and lower limit of integral in battery available energy calculation (see Fig. 2).
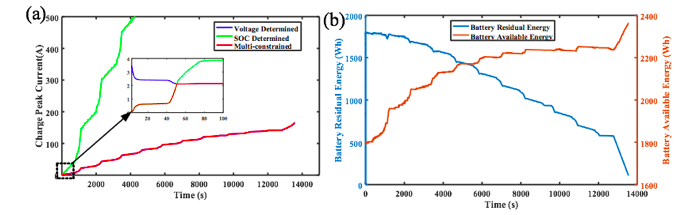
Fig. 2 (a) Battery pack peak discharge current; (b) battery available and residual energy. (Image courtesy of Chen Zonghai)
This paper is described in the article entitled A novel method for lithium-ion battery state of energy and state of power estimation based on multi-time-scale filter, recently published in the journal Applied Energy. This work was conducted by Xu Zhang, Yujie Wang, Ji Wu, and Zonghai Chen from the University of Science and Technology of China.
References:
- Vikström, Hanna, Simon Davidsson, and Mikael Höök. “Lithium availability and future production outlooks.” Applied Energy 110 (2013): 252-266.
- Mamadou K, Delaille A, Lemaire-Potteau E, et al. The state-of-energy: A new criterion for the energetic performances evaluation of electrochemical storage devices[J]. ECS Transactions, 2010, 25(35): 105-112.
- Dong G, Zhang X, Zhang C, et al. A method for state of energy estimation of lithium-ion batteries based on neural network model[J]. Energy, 2015, 90: 879-888.
- Zhang X, Wang Y, Liu C, et al. A novel approach of remaining discharge energy prediction for large format lithium-ion battery pack[J]. Journal of Power Sources, 2017, 343: 216-225.