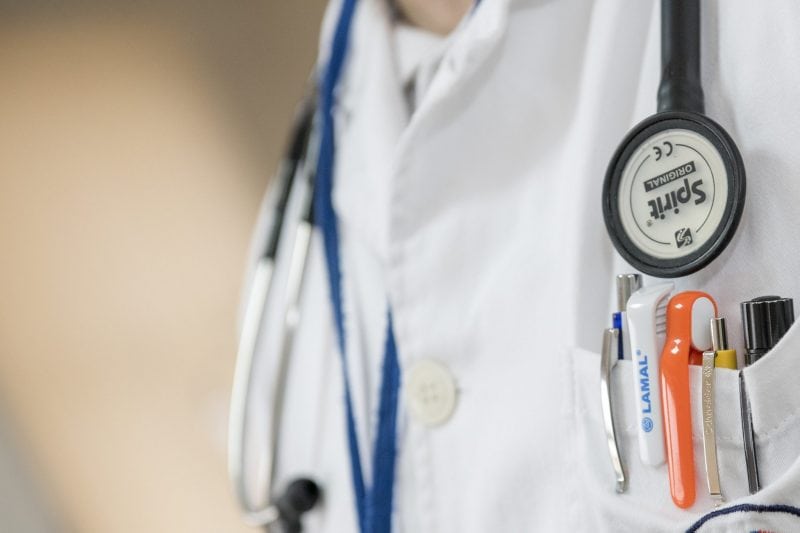
When drugs or devices enter the market, we want to be sure that there is effective, rapid post-market surveillance of adverse events in order to understand the risks attached to these products.
In this paper, we show how our patented Boomerang NLP technology, a blend of natural language processing and machine learning techniques, can effectively extract both common and rare events associated with particular devices from the MAUDE database, a real-world evidence repository, which is updated weekly. This characterizes the risk profile of a medical device much more quickly than currently-available measures.
Clinical trials results, where available, are seldom directly transferable to the post-approval environment, due to differences in population size, the expertise of practitioners, and patient composition. Most medical devices are not tested in clinical trials before being used by patients.
Products can have severe, often unexpected adverse events, causing patient injury. Numerous medical devices over the last five years have been recalled, voluntarily pulled from the market, or have been the subject of major litigation. These include synthetic meshes, hip implants, contraceptive devices, morcellators, and many others. Quickly obtaining and understanding post market information on unexpected adverse events, product problems and comparative effectiveness is vital for patient safety and optimal clinical decisions.
Patient registry information, a curated, tracked repository of clinical information, is considered the gold standard of post-market surveillance. However, registries can be expensive and cumbersome to set up and are only available for a very limited number of medical devices. Most importantly, dissemination of their information sometimes occurs months or even years after the procedure date.
As part of its effort to monitor post-approval complications with medical devices, the FDA employs the Manufacturer and User Facility Device Experience (MAUDE) system which is updated weekly. Device manufacturers must submit a medical device report (MDR) to the MAUDE database for every device-related serious injury, death or malfunction. Healthcare providers, patients, and consumers also can submit medical-device reports to MAUDE on a voluntary basis. The FDA receives several hundred thousand MDRs on serious injuries, malfunctions, and suspected device-associated deaths annually, and the reports are available to the public.
The MAUDE database contains massive amounts of timely information drawn from a broad group of contributors including narrative reports from doctors, nurses, patients, and family members. It contains data on tens of thousands of devices. As a result, it can be a rich source of information on device performance and outcomes post-market.
The sheer size of this data source, however, creates a challenge, as does the inherent “messiness” of the metadata and narratives. Inconsistencies are common in the manufacturer and device name fields; individuals supply this information, leading to wide variation in how a specific manufacturer or device is referenced across reports. For example, a single device was found spelled five different ways (Saien, Sapient, Sapaien, Sapiien, Sapien) in report metadata.
Our idea was to see whether the timely data available in the MAUDE database could anticipate what would be available much later in the patient registry data. We tested our results against two cases where the current gold standard of registry information is available, the Transcatheter Valve Therapy (TVT) registry for data on transcatheter aortic-valve replacement (TAVR) and transcatheter mitral valve repair (Mitraclip)
To rigorously compare the overall agreement between MAUDE- and patient registry-derived event rates, we performed a regression analysis comparing all events compiled from both the TAVR and Mitaclip MAUDE reports (based on the NLP analysis) to results from two registries.
We found a high correlation between our results and those of these patient registries. The proportional event rates reported by the Boomerang NLP analysis of MAUDE were relatively similar statistically to the event rates reported in the patient registries for both TAVR and Mitraclip procedures. Our regression analysis of TAVR (0.86), Mitraclip (0.77) and combined (0.78) indicates a high level of correlation across the TVT and Maude datasets.
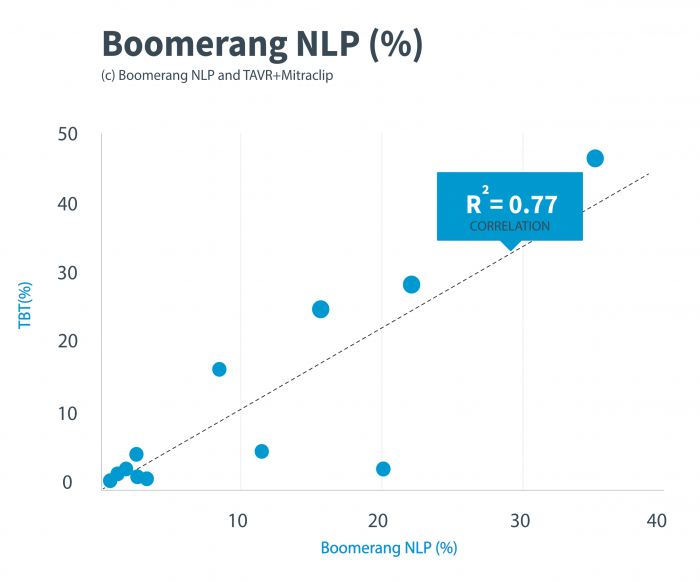
Figure 1. Regression analysis for the comparison of adverse events and device problems for TAVR and Mitraclip procedures between the TVT and Maude results. TAVR indicates transcatheter aortic valve replacement; TVT, transcatheter-valve therapy registry. Credit: Libbe L. Englander
We also conducted a t-test to compare the outcomes between each database and found them statistically similar. For both the TAVR and Mitraclip comparisons, virtually all event rates were not statistically significantly different from one another, except for bleeding-event rates.
We also did a direct comparison of specific outcomes. These included both common and rare events. For TAVR these include Perivalvular leakage, heart block requiring pacemaker insertion, bleeding, stroke, coronary occlusion, and valve embolization. For MitraClip, these included severe residual mitral regurgitation, bleeding, cardiac tamponade, stroke, single-leaflet device attachment (SLDA), and device embolization. Except for bleeding, which we find consistently underreported throughout MAUDE, none these outcomes were statistically different between our MAUDE analysis and registry information.
As a result, our analysis allowed identification of events specific to a certain device within a device class using MAUDE data. For example, the increased rate of pacemaker insertion seen in the MAUDE dataset was similar to the increased rate of pacemaker insertion noted during the same period in the TVT registry.
Not only did we detect common event rates, but we also highlighted the occurrence of more rare and life-threatening device-related complications, such as device embolization and coronary occlusion with TAVR, and single leaflet detachment, cardiac tamponade, and device embolization with Mitraclip.
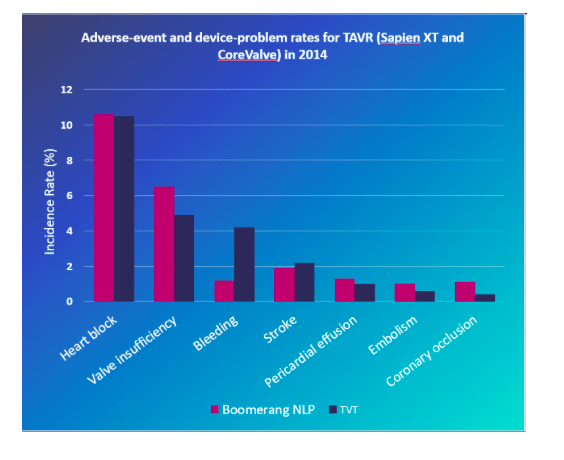
Figure 2. Adverse-event and device-problem rates for TAVR (Sapien XT and CoreValve) in 2014, comparing the MAUDE analysis and the TVT registry. Credit: Libbe L. Englander
By demonstrating correlation between the MAUDE-dataset event rates and the published TVT registry event rates for TAVR and Mitraclip procedures, we showed that the event rates abstracted from MAUDE correlate well with the real-world gold standard data for these procedures.
Since MAUDE data is publicly available shortly after the event occurrence, the use of NLP to sort through the data permits rapid and early dissemination of specific information related to events occurring as a result of device failure. NLP could thus have a key role in the post-market surveillance of newly commercially approved implantable devices. Using publicly-reported data that is widely available to demonstrate device-related complications could allow the FDA, device companies, providers and payers to learn of possibly dangerous issues with a particular device years before registry data is published.
Currently, there exists no NLP system to our knowledge within the FDA or industry to assist with post-market review of medical device MDRs or complaints. However, the use of NLP to process MAUDE data could further allow rigorous post-market surveillance on devices for which an established registry is not currently available.
This timely knowledge would allow regulators, clinicians, and device manufacturers to make rapid changes to prevent future events and patient injury or even death. This has important implications as a resource-efficient complement to existing registry structures and as a novel post-market data source when other active surveillance networks are unavailable or cost prohibitive.
There are also important public-health implications as NLP may improve the ability to perform rapid and reliable post-market surveillance of all reported implantable devices. An NLP strategy allows the FDA, device manufacturers, and the public to learn of rare events associated with device failure or malfunction that might otherwise not be reported for years.
These findings are described in the article entitled Comparison of Adverse-Event and Device-Problem Rates for Transcatheter Aortic Valve Replacement and Mitraclip Procedures as Reported by the Transcatheter Valve Therapy Registry and the Food and Drug Administration Post-market Surveillance Data, recently published in the American Heart Journal. This work was conducted by Benjamin Z. Galper from the Mid-Atlantic Permanente Medical Group, and David E. Beery, Gregory Leighton, and Libbe L. Englander from Pharm3r.