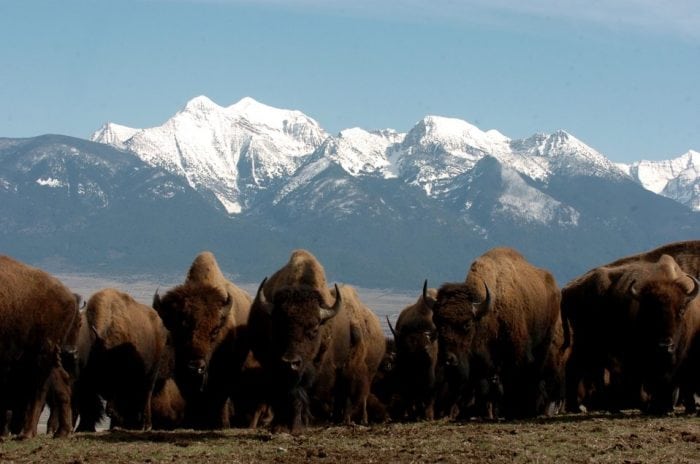
In 1945, an ecologist named Lee R. Dice was studying the association of different species of plants. In order to quantify the rate of association and to deal with the fact that one species may be dependent on another but the relationship may not be reciprocal, he introduced an index which he called the coincidence index. Since then, this index has appeared in the literature multiple times, and today it is most commonly known as the half-weight index.
As Hal Whitehead points out in Analyzing Animal Societies, the half-weight index and several other tools for measuring cooccurrence have been adopted by animal behavior researchers from the world of plant research “without much consideration for the implications.” Over the past three decades, a number of animal researchers have worked to consider these implications and develop measures of animal behavior which can be used as the basis for social network behavior.
Weko’s paper builds on recent work by Hoppitt and Farine to establish a framework for developing unbiased measures of association. Weko notes that association is a behavior which individual researchers define in their study of animals. The definition of association may be based on proximity, co-participation in activities, or direct interaction. Regardless of how the association is defined, it is important that when estimating the frequency of association that the estimates be unbiased. That is, there cannot be systematic differences between the way the data is collected and the way that the estimates are produced.
Qualitatively, these issues have been well understood by animal behavior researchers for decades. Factors such as the way a subject population is observed, the amount of the subject population that is observed, and the potential for individuals to hide or be misidentified have all been considered. This work formalizes the steps of data collection to dissect the sources of systematic variation in our data. This allows researchers to create unbiased estimates.
Additionally, Weko combines three statistical properties to make the task of developing custom association indices easier. He does this by observing that each data sample can be thought of as coming from a multinomial distribution. That is, as if each observation is the result of rolling a complex and fancy dice. This fact makes it possible to find maximum likelihood estimators without having to use calculus and, thus, greatly simplifying the task of making custom indices to handle special situations.
Finally, Weko shows that not all possible models of social behavior can be estimated. Simply because a set of behaviors can be described in nature does not mean that an unbiased estimator can be created from the data traditionally collected. While this is bad news for retroactive studies, it is promising for future studies. By developing estimators to meet the specific needs of the situation, researchers can identify the way that they need to collect their data.
These findings are described in the article entitled Isolating bias in association indices, recently published in the journal Animal Behaviour. This work was conducted by Colonel Charles W. Weko from the U.S. Army.
* The views expressed in this article are those of the author and do not represent Department of Defense policy or Army regulation.