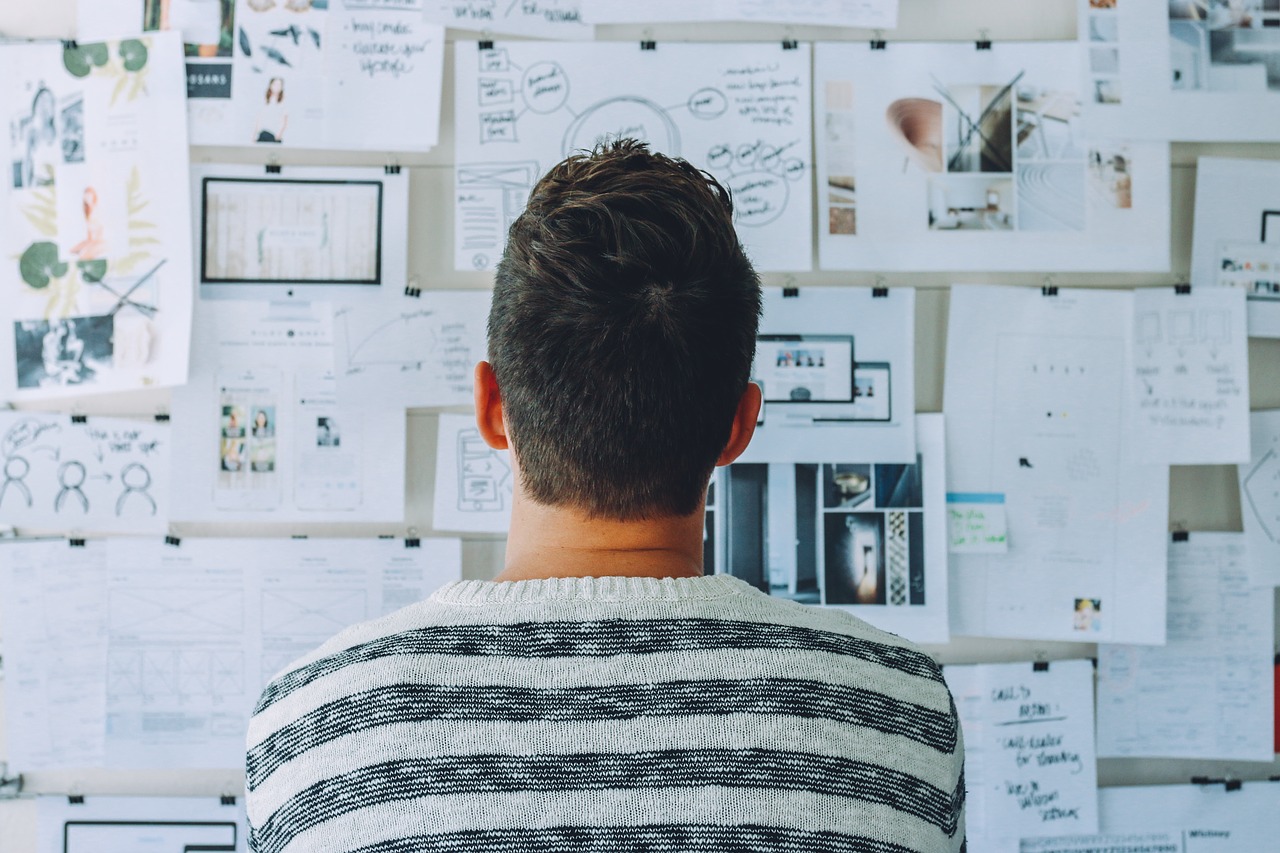
That the mind is comprised of distinct systems is one of the earliest and most recurring ideas in psychology. Plato claimed the psyche is comprised of three parts: reason, appetite, and temper. Over 2,000 years later, Sigmund Freud (in)famously defended a view of mental life as being comprised of the id, ego, and super-ego. Recently, Nobel Prize winner Daniel Kahneman’s best-selling book, Thinking, Fast and Slow (2011), centered on the claim that there are two main cognitive systems: System 1 is fast, automatic, emotional, and unconscious; and System 2 is slow, controlled, logical, and conscious (Table 1).
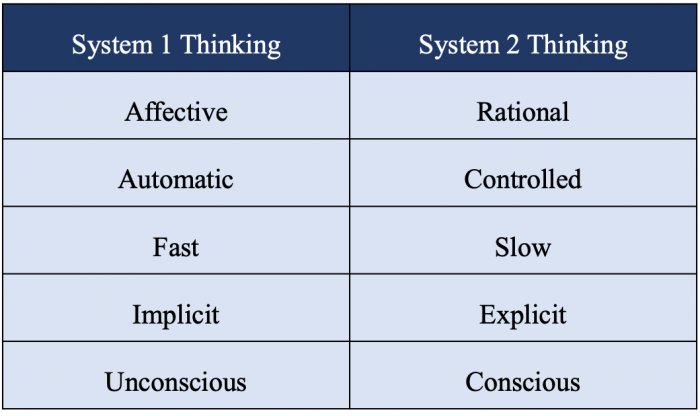
Table courtesy Luis H. Favela.
The distinct systems view fits nicely with the computational theory of mind (Rescorla, 2017). Since the mid-1950s, much of psychology — as well as cognitive psychology, cognitive science, and neuroscience — have been committed to the general idea that minds are computers: brains are the hardware, and thinking is the software. From this approach, thinking is a kind of computing that involves rules (such as math: 2 + 2 = 4) and representations (such as concepts: “D-O-G” = four-legged, mammal, licks faces, etc.), and is typically executed in a linear or sequential manner. One of the main jobs of psychologists has been to identify the mind’s organization in order to build a list, or taxonomy, of various systems (Anderson, 2015; Poldrack & Yarkoni, 2016).
Another feature of the computational mind is its modular organization. Like a computer with hardware and software that processes specific kinds of data, so too does the mind have a set of modules that process only some kinds of information. For example, visual modules process light and language modules process particular sounds. Notice that visual modules do not process sounds and language modules do not process light. In that way, modules are “informationally encapsulated,” that is, they are cut off from each other.
Luis Favela and Marieke van Rooij claim that reasoning is incorrectly understood as falling within two distinct kinds of processes. Instead, they argue that nonlinear dynamical systems theory (NDST) can provide a fresh perspective on reasoning.
NDST is a branch of dynamical systems theory (DST). DST utilizes mathematical tools — such as calculus and computer simulations — to model and understand the ways systems behave over time. Nonlinear systems are those whose outputs are not proportional to their inputs. Such nonlinearities can result in systems having major qualitative shifts in their states. For example, the relationship of temperature and pressure to water is nonlinear in that at certain temperatures and pressures a collection of H2O molecules can suddenly shift from liquid to solid or gas. Still, though there is a drastic change from a liquid to a gas, the systems are still comprised of the same H2O molecules. Similarly, Favela and van Rooij argue, is the case with reasoning.
Although there are major shifts between different kinds of reasoning processes — such as fast and slow, emotional and logical, etc. — it does not follow that the shifts are among isolated reasoning modules. In other words, fast reasoning is one kind of information that cannot be processed by slow reasoning modules and vice versa. What then is the fresh perspective NDST provides this issue?
Favela and van Rooij argue that the apparently different kinds of reasoning do not occur via isolated modules or distinct systems but, instead, occur along a continuum of reasoning capacities (Figure 1).
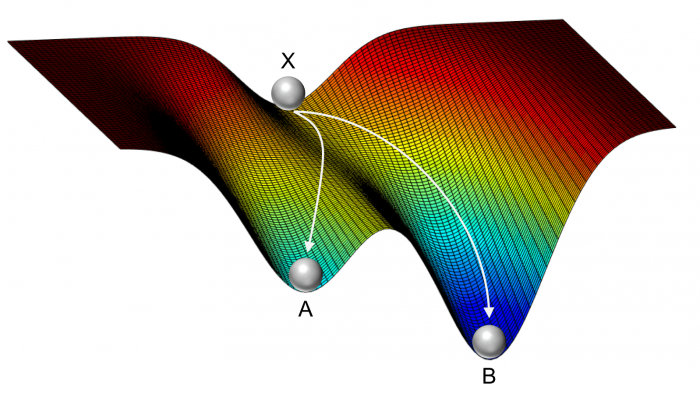
Figure 1. A landscape of reasoning capacities (Favela & van Rooij, 2019). A fast response (A) or a slow response (B) may occur based on different reasoning scenarios (X). Figure courtesy Luis H. Favela.
For example, the solution to a simple math problem (1 + 1 = 2) could be reasoned fast (A) or a more complicated math problem could be reasoned slow (137 + 48 = 185). Utilizing this approach, Favela and van Rooij reference their earlier experimental work on decision-making under conditions of uncertainty (van Rooij, Favela, Malone, & Richardson, 2013). In that work, they demonstrated that some types of reasoning styles — risk-seeking and risk-avoiding — that would be categorized as isolated from each other under the two-systems approach, could be manipulated and explained as falling along a continuum without a clear distinction.
In addition to explaining how NDST can be applied in the psychological sciences and providing an example, Favela and van Rooij suggest directions for future research that can continue to investigate reasoning and other cognitive processes, not as isolated from each other, but as falling along a landscape of continuous capacities.
References:
- Anderson, M. L. (2015). Mining the brain for a new taxonomy of the mind. Philosophy Compass, 10, 68-77.
- Evans, J. St. B. T., & Stanovich, K. E. (2013). Dual-process theories of higher cognition advancing the debate. Perspectives on Psychological Science, 8(3), 223-241.
- Favela, L. H., & van Rooij, M. M. J. W. (2019). Reasoning across continuous landscapes: A nonlinear dynamical systems theory approach to reasoning. Cognitive Systems Research, 54, 189-198.
- Kahneman, D. (2011). Thinking, fast and slow. New York, NY: Farrar, Straus and Giroux.
- Poldrack, R. A., & Yarkoni, T. (2016). From brain maps to cognitive ontologies: Informatics and the search for mental structure. Annual Review of Psychology, 67, 587-612.
- Rescorla, M. (2017). The computational theory of mind. In E. N. Zalta (Ed.), The Stanford encyclopedia of philosophy (spring 2017 edition). Retrieved May 23, 2019 from https://plato.stanford.edu/archives/spr2017/entries/computational-mind/
- van Rooij, M. M. J. W., Favela, L. H., Malone, M. L., & Richardson, M. J. (2013). Modeling the dynamics of risky choice. Ecological Psychology, 25, 293-303.