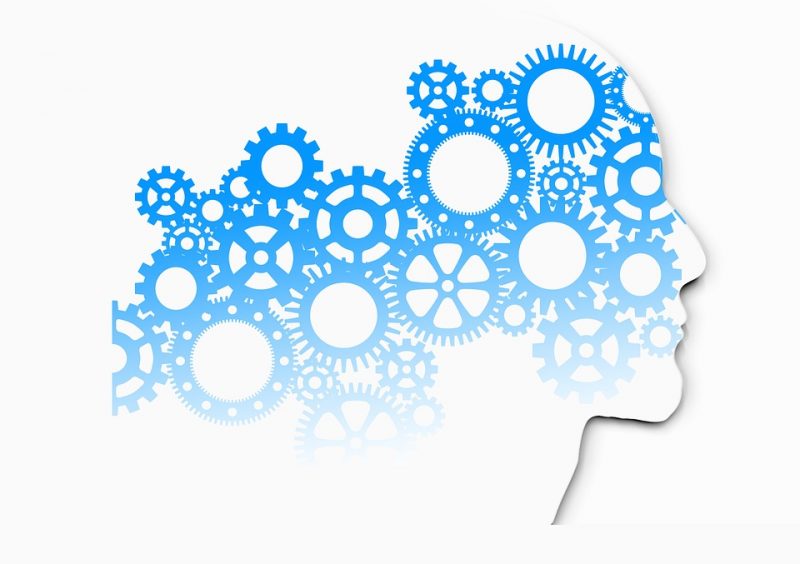
It is interesting to see how the popularization of neuroscience has led to the use of funny expressions like, “This is too much for my brain right now,” “That girl has a big brain,” “My brain/mind can’t take it anymore,” or “My mind feels foggy.” This is funny because these expressions imply some sort of separation between brain, mind, body, and self.
While we like and popularize these terms – both because they sound funny and because of influences of dualism still among us – neuroscience has known for many decades that there is no such separation. You are your brain (in motion)! What we usually mean by the popular use of “personality” and “mind” all come from the dynamics established in our brains. Therefore, if our brains change, we change! Our personality traits may change, and so may what we understand as us!
Understanding what and how much would be altered in us if our brains were somehow modified is exactly one of my long-term goals in life. However, this kind of prediction requires understanding some rules of the dynamics in the brain. Two features of the brain that eluded neuroscientists for decades are key to achieving this understanding: functional segregation and integration.
Functional segregation means that the brain has areas specialized in certain functions. Some call it localizationism. These functionally segregated areas are highly integrated among themselves, allowing different brain functions to coordinate. The perception of who we are and everything we do pretty much result of this big functional integration. When scientists say the brain is a network, actually, it is a network of networks. It is a highly integrated network of smaller, specialized networks of neurons. The bigger network integrates (and coordinates) the functions to which the smaller networks give rise.
In the last decade or two, a branch of mathematics called Graph Theory, or Network Science, started being incorporated into neuroscience and has been changing the field enormously for allowing to understand the brain’s network organization and dynamics like no other. Briefly, among many other things, it allows assessing, quantifying, and understanding the segregation and integration in the brain based on measures of connectivity among neurons and brain regions. If a certain cognitive function requires certain brain dynamics, we can characterize that network. Then, in an organism that has a brain alteration, we can verify how that alteration still allows the characterized brain dynamics to occur or whether the brain just finds “alternative” dynamics to perform that cognitive function – and, therefore, the behavior.
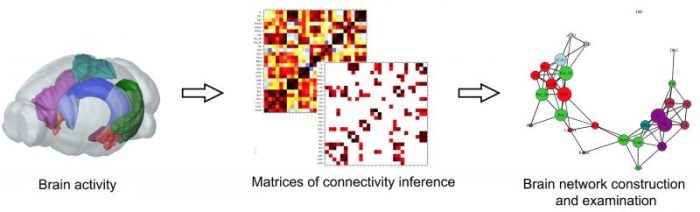
Credit: Cesar Coelho
In our recent work, published in PLOS Computational Biology, that was exactly the approach taken. We were trying to understand the importance of a region called the hippocampus to brain dynamics established during learning and memory. We know the hippocampus is important for an umbrella of functions related to learning and memory, but there are certain kinds of information and specific conditions in which lesions to the hippocampus do not result in memory impairments. We submitted rats with hippocampal lesions and control rats to a certain learning paradigm in which the rats with lesions were not impaired.
We wondered what dynamics are established in the brain absent from the hippocampus that still allow for this learning, given that we know that the hippocampus has a participation in that learning when it is intact. We looked into the rats’ brains, characterized their brain connectivity networks, and started making comparisons that could lead to an explanatory hypothesis for the learning in the rats with lesions.
First, we observed that the lesion did not change the overall interactivity of the brain. When we measured the segregation and integration of the regions that had their activity tracked, we saw no difference. That was interesting because it basically shows that damaging one region does not necessarily disrupt the overall information exchange occurring after learning, which is a valid initial hypothesis.
Second, we calculated different metrics of centrality (importance) for each brain region in our network and identified the regions called “network hubs,” meaning regions that display a high importance according to most of the metrics used (3 out of 4). The nice thing about this is that these metrics capture different concepts of importance. For instance, the degree is defined by the number of connections a region possesses, capturing the idea that “the more connections, the more important” a system is. Betweenness captures how much a given region is necessary to connect any two other regions, and Eigenvector centrality measures how much the important regions connected to other important regions. What was interesting at this point was that the regions identified as hubs in the control group were different than the ones identified in the lesion group, suggesting that, in the absence of hippocampus, the interactions can change so support learning.
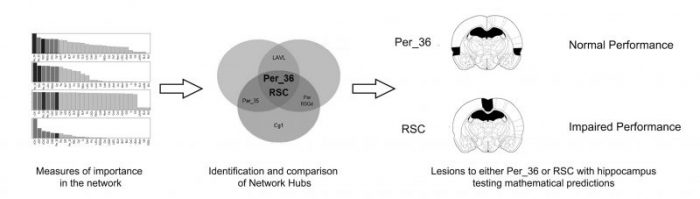
Credit: Cesar Coelho
When we actually compared the centrality values of each region between the groups, we observed that the hubs identified in the lesion group, in fact, had significantly higher centrality values when compared to the control group. These regions with higher centrality in the lesion group were the Retrosplenial and Perirhinal cortices. Both regions are well known to have intimate connections to the hippocampus and to be involved in learning and memory as well.
In a parallel analysis, we also compared the inferences of connection between the groups and identified interactions that increase and interactions that diminish in the lesion group. Important to say that these change in interactions were not related to the hippocampus, revealing the dynamic effect that the hippocampus has in the interactions of other regions of the network.
Finally, we tested experimentally the validity of these mathematical calculations. In another cohort of animals, we made double lesions of the hippocampus and one of the “new hubs” (retrosplenial or perirhinal cortex) and submitted them to the same learning and memory task. We observed that lesion to the Hippocampus together with Retrosplenial cortex led to a drastic learning impairment, which was not observed in control groups with single lesions of the hippocampus or Retrosplenial cortex. The same event was not observed in double lesions involving the Perirhinal cortex.
These results made a lot of sense to us since the Retrosplenial cortex was the region with the highest centrality values in the lesion group and presented the most robust differences when compared to the control group. Besides, it can be the case that the Perirhinal cortex affects the learning just as the hippocampus – only given certain conditions – and not dismantling the network enough to prevent the learning to occur.
If one asks me what it means, I would say that it seems that in the absence of an important region (route of information processing), the brain reacts by increasing interactivity in the functionally closest region to that damage (maybe even overloading it) in order to perform the cognitive function as well as possible given the circumstances. We actually got excited when we saw converging evidence from papers in humans showing the Retrosplenial cortex had the same increase in connectivity after traumatic brain injury (which can deplete some functions temporarily). Our hypothesis is that we were successful in blocking the formation of a minimal structure of interactions that the network needs to establish so learning occurs.
We are currently doing two follow-ups to this study. In the first, we are evaluating whether these lesions, impairing learning or not, change the content of the information being learned, which requires more ingenious and complex behavioral tasks. In the second, we are evaluating what happens to the network when learning fails. We are asking what is the minimal structure of interactions in the network necessary to promote learning – and performance.
These findings are described in the article entitled Network supporting contextual fear learning after dorsal hippocampal damage has increased dependence on retrosplenial cortex, recently published in the journal PLOS Computational Biology. This work was conducted by Cesar A. O. Coelho, Juliana C. Kramer-Soares, and Maria Gabriela M. Oliveira from the Universidade Federal de São Paulo, and Tatiana L. Ferreira and João R. Sato from the Universidade Federal do ABC.